It seems quaint to think about now, but there was once a time in software engineering when software was developed, shipped, and then it was just… done.
“Software used to be a single artifact that didn’t need to be maintained as a running system,” Census founder and CEO Boris Jabes said. “A lot of what engineers and data teams do now is just ‘keep the lights on’ – it’s just making sure the dashboard doesn’t go down.”
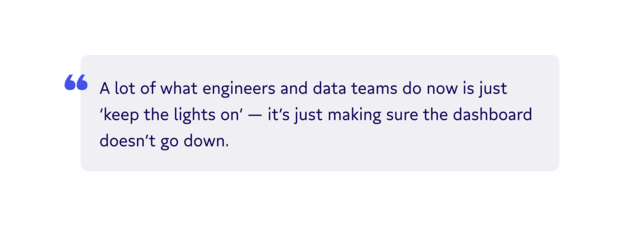
Today, almost all software operates in a live environment where it constantly evolves and updates. In the same vein, we now live in a data-driven world where data is in a constant state of evolution and is never truly “done.”
Egor Gryaznov, co-founder and CTO of Bigeye, said it was not so long ago that software updates came out every six months or so. Now, patches and updates are released monthly, if not faster.
“[Years ago] a data warehouse was a piece of software you installed on your server,” he recalled. “That was a node, and you created more nodes that you had to manually manage. Because there was so much complexity in this, the software had to move slower.”
As the evolution of software has picked up speed, it has carried everything else along with it. Once upon a time, it was enough for data teams to simply run a single monthly report. Today, data analytics and data science have advanced to the point where, like software engineers, data teams are working in a live environment.
“The infrastructure can move faster now,” Egor continued. “But because the infrastructure can move faster, everything else can move faster. Now data is exploding – and it’s breaking at an even faster rate.”
So much data, so little understanding
As data-driven business has matured, the ability to collect and store data has too, thereby changing the original question from, “Can I get this data?” to “How do I curate and sift through this data?” Organizations are buried under a deluge of constantly updating data sets – not all of which are useful to the decision-making process.
Bigeye is a data observability platform developed to help people sift through their data and make it work effectively, so customers can make informed decisions. Initially, Egor said, many customers can’t even articulate which data sets are most important to them.
“That’s going to be one of the most interesting challenges over the next couple of years,” he said. “There’s a lot of information you can collect, and there’s a lot of information you can apply. Which information is important, which is correct, and which should you be using?”
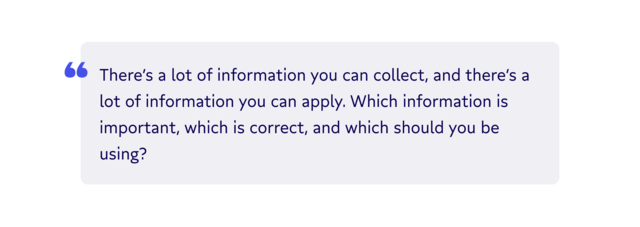
People outside of the data team will often ask for reporting on short timelines simply because they can, Boris said. They might ask for a report to run on Tuesdays and Thursdays at 3 pm, though the information gained then is no more important than it would be if it were obtained at any other time. Teams will run an ETL every hour just because the system can support it, not because it’s what is best for the system – or the people relying on the data for that matter.
“You have all this data updating 24 times a day for a nightly report that’s never looked at again,” Egor said. “We need to start building systems that are optimized for how important the information is to the data consumer then use that knowledge to make more efficient use of infrastructure and time.”
Ideally, queries should be sorted on categories of urgency, Boris said. That gives the data team flexibility to run them at the optimal time for the system and have the data ready when the receiving humans need it.
“Running things on a chron inferred from people’s desires is one part,” Egor agreed. “Another part is, what is the optimal way to run this given the current state of the database or warehouse? Should I batch them into queries? Should I run one large query? Should I wait because the warehouse right now is overloaded? There’s a lot of interesting potential here.”
Knowing what to measure
As the types of data expand and the sheer volume of data explodes, data catalogs will have to evolve with it, Egor said. Ideally, a data catalog is a central store: it identifies what the data is, what it looks like, what its use cases are, who knows about it, and what state it’s in. The next level, he said, will filter the data into “the stuff you care about” and “the stuff you don’t care about,” revamping the customer experience entirely.
In a growing number of companies, data is influencing user experience in real-time. When it works, this is a competitive advantage; but when it breaks, issues have to be resolved quickly. The pressure to maintain data quality in a live environment is a key factor igniting the growth of data observability and visualization.
Egor recalled working for Uber around the time the company implemented its first data warehouse in the late 2010s – though it wasn’t enough.
“All this data was in a central place. We were using it for all this reporting and all these analytics, but it broke all the time,” he said. “That’s when you start thinking about reliability and quality.”
At one point, he said, a bug forced the data team to close off the data warehouse for a week. Instead of making queries of the database, other departments had to send queries to the data team, who would run them as quickly as possible while they simultaneously wrestled with getting the warehouse corrected.
“Why does data quality matter? Because if we knew there were a bunch of nulls in this one table, it would have raised red flags earlier,” he said. “But we were so overwhelmed with the rest of the infrastructure we didn’t have monitoring on this.”
As the technology to ensure data reliability continues to mature, it will become even more important for companies to integrate data-driven decision-making. They’ll need to be selective about which data is being analyzed and how that analysis impacts business intelligence and, ultimately, business decisions. Those willing to move fast and change their practices will have a strategic advantage over those taking a slower, more cautious approach to analysis and change.
“I think there’s a lot of interesting information you can figure out about what data is important, when it is used, and how it is used,” Egor said. “That information should guide when to load it and when to check it.”
Want to learn more? Listen to the full conversation between Boris and Egor below or on all your favorite streaming platforms. 🎧
Got thoughts and opinions about this topic? Join the conversation around this, and many other data best practices, in The Operational Analytics Club.