If you’ve been in the data world (or, let’s be honest, the real world), you’ve probably noticed just how homogeneous everything feels. And if you’re a hiring manager building a data team, an individual contributor on a data team, or just wandering the conference floor of most industry trade shows, you’ve probably experienced this homogeneity firsthand in the data industry.
Now, finally, diversity is a hot topic – and rightfully so. But while everyone seems to agree we need more diversity in data, we don’t necessarily agree on how to get it. To dive deeper into this topic, we hosted a keynote session titled, Diversity in data: Modeling a better future for data teams during this year’s Summer Community Days.
It featured some inspiring data professionals who have an insider’s perspective on the diversity challenges talking about the diversity problem in data, including:
- Julia King, VP of data and analytics at Carta
- Christine Ndege, contract data engineer
- David Jayatillake, head of data at Metaplane
- Jessica Cherny, senior data analyst at Fivetran and founder of Data Angels
- Julie Beynon, head of analytics at Clearbit
- Meghan Cassidy, data scientist at Brooklyn Data Co.
This fireside chat amongst data professionals sparked a fruitful conversation regarding:
👐 What modern data companies can do to foster diversity in their teams
❗ Why diversity in data is something data teams (and companies) of all sizes should care about
📉 How a lack of diversity can negatively impact individual practitioner’s careers (and the teams they’re a part of)
🚪 The role of community in opening up doors for more diverse voices in our industry
Why it’s important to find people who look like you
To kick things off, Julie Beynon said as a young data pro, all her role models were men. 👨But by achieving a leadership position herself, she hopes to change that for the next generation.
“If you have to do more than one scroll on a company’s About Us page to find someone who looks like you, that can be pretty intimidating,” Meghan Cassidy agreed.
By now, we should all know that “diversity” is more than a buzzword. In fact, McKinsey data shows that companies with employees from a variety of backgrounds generate 20% more innovation, 35% better financial returns and 38% more revenue than those who don’t. 💰 Unique backgrounds lead to unique perspectives, and as important as that is in any business, it’s an even bigger deal in data.
In any given week, the data team might be customer-facing, business-facing, and product-facing. We might have both a rules-based text analysis project and a machine-learning model on our plates. So, the more unique backgrounds we have on the team, the better equipped we are to catch whatever the business throws at us.
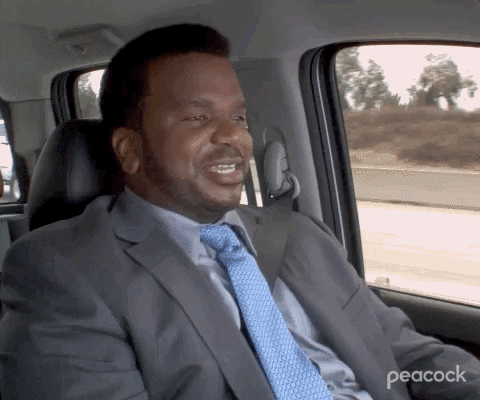
“There’s a lot of different facets to a data role,” said Julia King. “Different backgrounds, different skills, and different points of view make that multifaceted interface a lot better.”
Besides varying gender, racial, and ethnic makeup on our teams, we can also look at hiring employees from various career backgrounds. Different career backgrounds lead to different ways to view (and solve) problems, and you might need both viewpoints to come up with an innovative solution. And if we’ve learned anything in the past few years, it’s that we should evaluate candidates based on their skills and potential, not their job titles.
Diversity in data can’t just be an HR project
All the panelists agreed that for initiatives to make an impact, they have to start in the C-suite. Data consultant Christine Ndege said she’s seen hiring committees make an effort to recruit diverse candidates – but they can’t make it stick without executive buy-in.
“If your diverse candidate isn’t adequately supported, you won’t see retention,” she explained. “But if a manager thinks it’s important, the likelihood of success increases. The managers that actually make the effort to engage with diverse communities end up with more diverse teams.” 🤝🏽
Many leaders acknowledge the lack of diversity in the tech industry without trying to do anything about it, said David Jayatillake.
“They blame the funnel, or they say ‘This split is typical in our industry,’ and that’s where they leave it,” he said. “They need to say, ‘We’re going to come up with actions and spend money to solve this problem.’”
And David is taking the lead in this effort. For example, David said he convinced a company hiring for a position on an all-male team to spend on a recruitment agency to make sure 50 percent of the candidate pool was women. Today, the team is twice as big – and evenly split between the genders. 👨🏼👩🏾🦱
But investment doesn’t stop once candidates are on board. Mentorship matters. If there’s no one in the company who would make a suitable mentor for a new hire, companies can connect them with outside groups or agencies.
It’s also important for direct managers to recognize the pressure underrepresented employees put on themselves.
“If you are the only woman or minority on your team, you may not feel comfortable sharing struggles with your manager,” Jessica Cherny said. “You feel like you have to perform two times better than anyone else because you’re representing all of womanhood or all the people who came to a data career from a boot camp.”
How to bring more diversity into data
Retaining diverse employees is crucial – but before you even consider retention, you have to figure out how to recruit them. Our panelists had three main recommendations for improving recruitment:
- Look past your usual candidate pools 👀
- Encourage people from other career fields to transition into tech 👋🏻
- Remove gatekeeping requirements from job descriptions 🔓
1. Take a dip in a new candidate pool
When positions on data teams open, companies usually need to fill them fast. And when you need someone fast, you tend to go back to where you’ve found new hires before rather than exploring new avenues.
“You tend to lean on your network. And your network tends to look a lot like you,” Julie said. “To counteract that, when you’re not in a rush for hiring, work on building a more diverse network. Reach out to different communities, different networks, and different Slack channels. Then when you need to hire, you have a more diverse pool to go to.”
You might also limit yourself by always recruiting from the same college programs. If a bulk of your team shares the same one or two alma maters, that’s a sign it’s time for HR to visit some new campuses. 🎓
2. Encourage data as a second career
A lot of people without a tech background think data is intimidatingly complex. We can all help dispel that stereotype by talking about what we do and how we learned what we now know.
“I don’t have a formal education in data,” Julie pointed out. “I started in marketing and learned as I went. There are so many roles in data that require little technical skill to get started, and you can learn it all online. Then once you start learning you can become a little more technical every year.”
While new recruits do need some foundational skills, what we should really look for is a curious attitude and the ability to figure out unique problems. Problems in data are always changing, so even a candidate who has “done it all” will eventually come up against something new and need to work it out.
3. Get real with job descriptions
Job descriptions are supposed to do two things: Attract qualified applicants and discourage unqualified applicants. But sometimes, our panelists said, they go too far.
“What does ‘qualified’ mean?” David asked. “Do we really need someone with a degree in a STEM field? Why do we need that? When you start removing those gatekeeping elements of what makes a person ‘qualified’ to work here, you unlock a more diverse population.” 🔓
When writing job descriptions, pay attention to the relevance and depth of requirements. It’s fine to say applicants need to know a coding language, but do they really need five years of experience? Is there a reason someone with two years of experience couldn’t do the job?
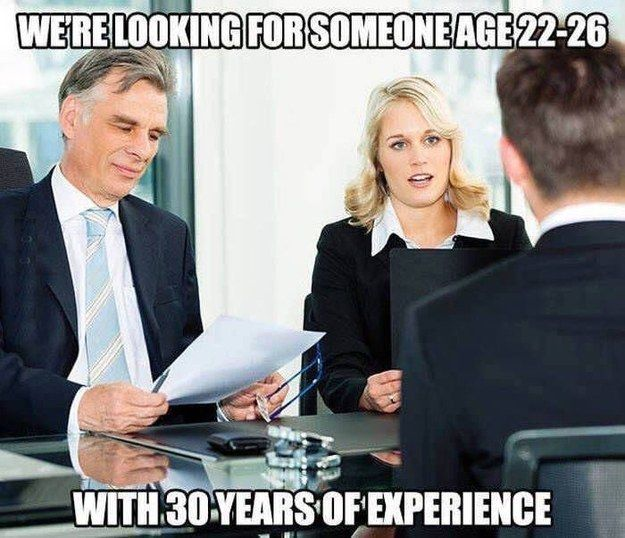
Julie said at Clearbit, she works with the content writers on the marketing team to make job descriptions enticing and inclusive. Leveraging those internal resources may take longer than throwing down some arbitrary experience requirements, but it yields a much richer field of applicants.
The future of diversity in data
Data, like a lot of tech fields, is still largely dominated by white men. Fortunately, though, the scales are now tipping toward balance as hiring initiatives of the past few years start to pay off. ⚖️
“I see a lot more women in leadership roles, which is amazing,” Julia said. “We definitely have a long road ahead of us, but I think we’re at least trending in a positive direction.”
David said he’s encouraged by the very fact that we’re talking about increasing diversity. Early in his career, he said, that wasn’t the case. After all, the first step in making change is actually wanting to make the change.
✨Watch the full panel discussion and more Summer Community Days talks here. Then head over to the Operational Analytics Club to share your view and join the conversation.