Hugo, a meeting productivity hub, is a one-stop shop for businesses to prepare for meetings, take notes, and simplify meeting workflows for entire teams, all while integrating with existing tools. Darren Chait, co-founder and COO at Hugo, leads the small but mighty team of 20 developers, marketers, product managers, and a recently added sales team.
In this interview, Darren shares how he went from being a corporate lawyer to setting up the modern data stack and about how Hugo is using data to provide a more personalized user experience for their customers, resulting in more conversions.
Tell us a little about your story, how did you learn about data and tools like dbt?
I started as a corporate lawyer, but in 2017, I founded Hugo with Josh Lowy, who’s now the CEO, because we were both frustrated with the state of meetings. When we started Hugo, I was more business-oriented than product-oriented, which meant much of my focus was on go-to-market.
Thanks to the no-code movement and the massive strides that allow non-technical individuals to build solutions, I’ve been able to dive deep into data engineering, data analytics, and building out the data layer for Hugo. With a little bit of basic SQL, I’ve been able to build a complete ETL layer and respond to requests every day. I started as a corporate lawyer – and now I’m building out complex data stacks thanks to products like dbt and Census.
At Hugo, we initially started by using dbt Cloud. Going in, I didn’t have much experience, so a contractor and a member of our growth team showed me the ropes. But, ultimately, as time went on, I used my own knowledge of SQL (plus some other tools like Metabase and Chartio) to navigate.
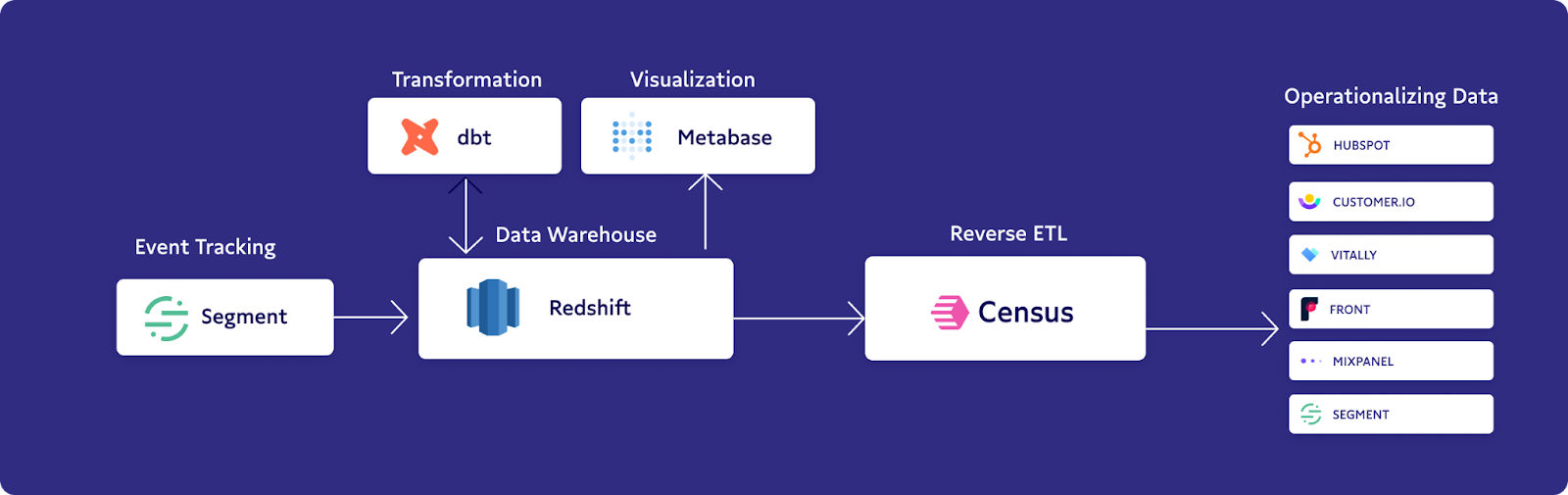
What’s the data culture like at Hugo?
So, culturally, we're a very data-savvy organization. Even from the early days when we only had a handful of people on the team, we've always been focused on data. Because of this, we saw an opportunity to take action with our data and analytics to provide a better user experience for our customers, instead of just for informational purposes
Next up, we asked Darren to deep dive into an impactful operational analytics use case with us.
Show & Tell: Increasing conversions 3-4x by syncing email personalization data to Customer.io
At Hugo, we rely on Customer.io as our email service provider which allows us to send millions of emails each month to our users. We've always had analytics data coming to Customer.io through Segment, but there, we didn’t have the ability to transform that data. For example, I could say, “When this event happens, send this email,” or “If this event happens five times, send this email,” but I couldn't do anything with that raw data for our emails.
Inserting this raw data was important to us because we wanted to personalize our emails. With it, we could show our users the top 10 people they meet with the most, as well as weekly summaries of their activity. When we realized we couldn’t do this with Segment on its own, we connected Census to run queries on our data warehouse and deliver the transformed data back to Customer.io to trigger personalized emails.
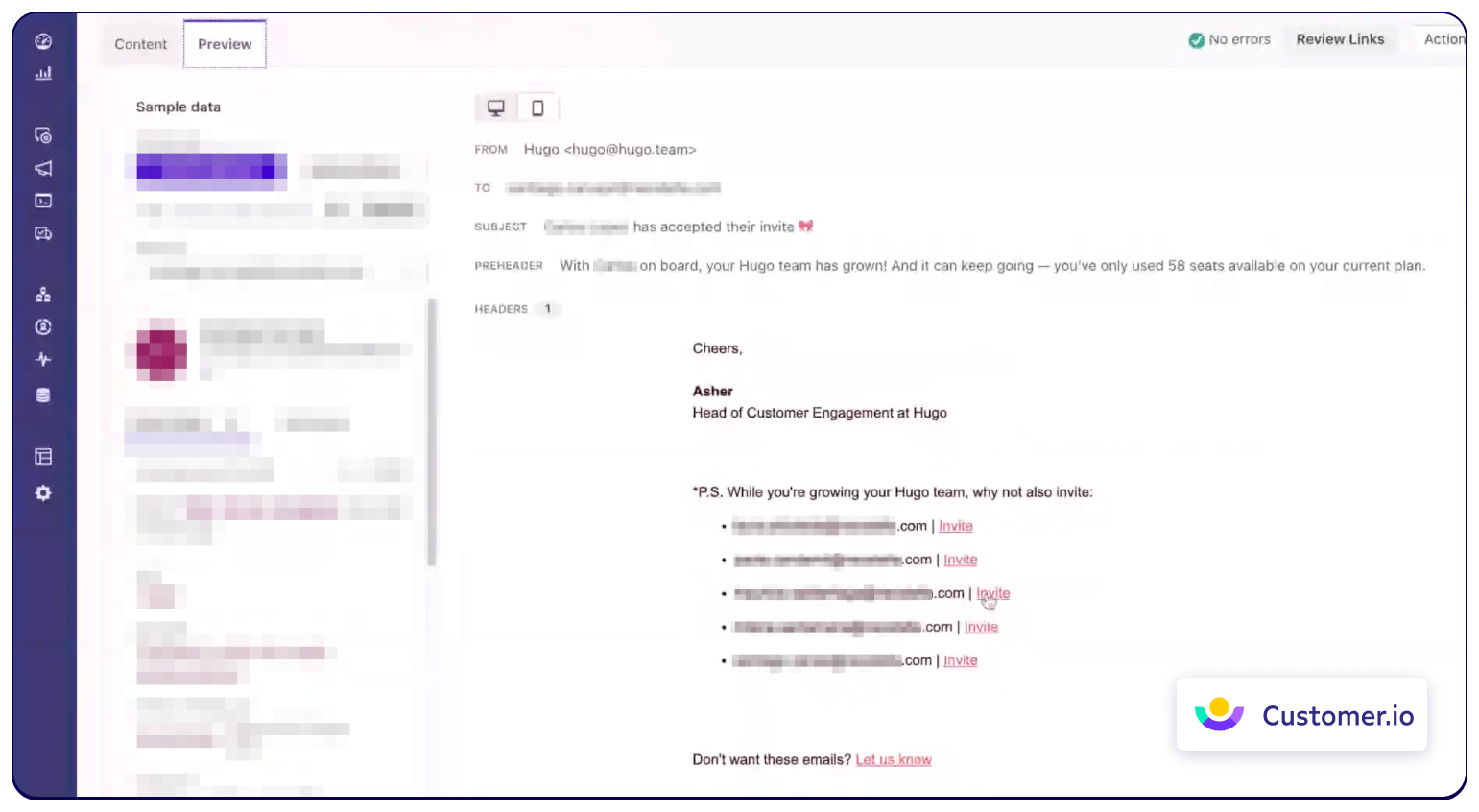
Early engagement scoring to drive activation
Another use case we wanted to create was an early engagement score. We conducted some market research and learned that what users do in their first seven days is predictive of long term retention. So, we decided to create a model to calculate this score with a basic query that returns whether the trait of “early engagement” is true for a particular user. Then, we can sync this information with Customer.io which shows whether these users are engaged.
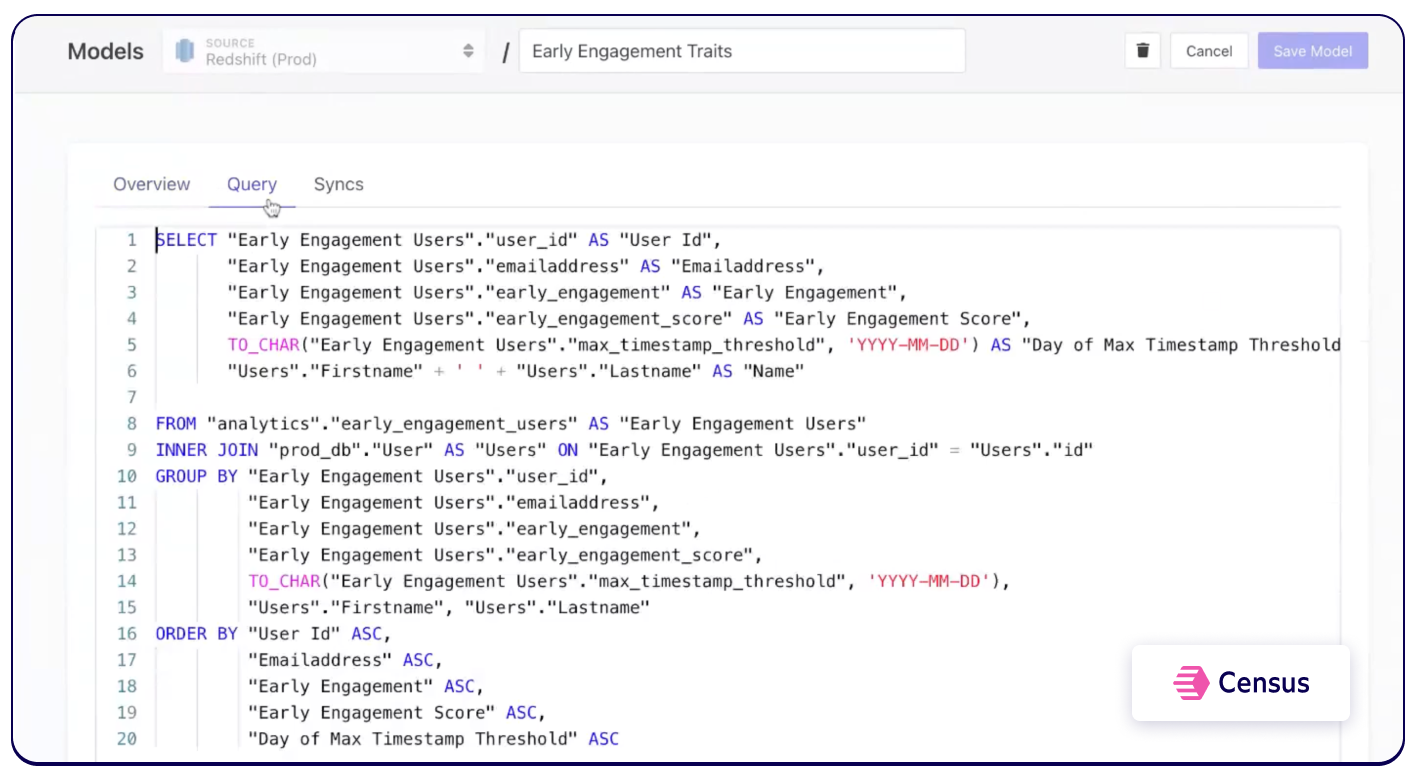
Every day at 6:00 PM, we update traits for that day, affecting which emails users are going to receive. If they're already engaged, they’ll receive messages about upgrading, as well as encouragement on how to take advantage of certain features. If they’re not yet engaged, we’ll send different emails that focus on getting them activated.
What’s been the impact of up-leveling your approach to data for the business?
Overall, we’ve gotten an immense amount of value from up-leveling our approach. One major benefit is that we haven’t needed to hire a data engineer, and we can still do a high level of transformation and integration ourselves.
Speed is a factor, too, so we love that we’re able to access and use our data quickly. Plus, access to data is now democratized, which means our marketers, product managers, developers, and others can jump in and get the data they need. Not only is it faster, but it also empowers the team.
The biggest benefit, though, is how data impacts the effectiveness of our campaigns. Highly personalized campaigns convert three to four times better than generic ones, so whether we’re suggesting that users jump into Hugo, inviting certain people, or specific integrations, we know those campaigns are going to perform – an absolutely essential factor for us as we grow.
What advice would you give to other startups looking to drive more impact with data?
The best advice I can give to teams looking to drive more impact with data is to get your stack right from day one. Whether you’re aware of it or not, there's a switching cost; every time you change things as you scale, it costs a lot of time and money, and it only gets worse and more complex because of the quantity of existing data as you mature. If you choose the right tools from the beginning, you’ll be better positioned for the future.
I also recommend warehousing everything. Having everything in one spot is crucial – even the best tools in the world won't do anything if your data's not in the right place. Carefully choose a data warehouse and make sure that everything's syncing to it.
Finally, we've seen success because, as a business, we believe data is for everyone. It's democratized and is accessible by everyone rather than owned by a siloed data team. This not only empowers our team but helps us deliver more value for our users, quicker. My advice is to think very closely about the culture you want to create around data – for your team and your business.
Want to be featured in our next Select Stars profile? Apply here and we’ll be in touch soon!