TABLE OF CONTENTS
How to find the true ROI of the modern data stack | Census
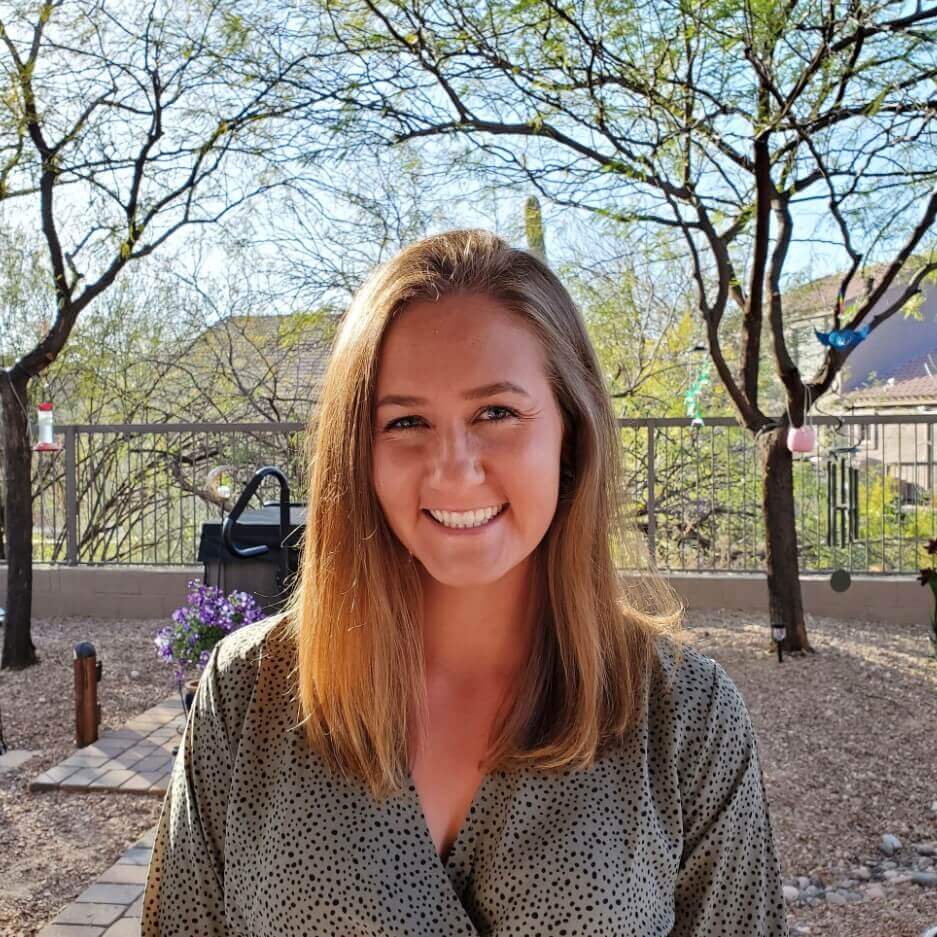
Nicole Mitich is the content marketing manager @ Census. She's carried a love for reading and writing since childhood, but her particular focus is on streamlining technical communication through writing. She loves seeing (and helping) technical folks share their wisdom. San Diego, California, United States
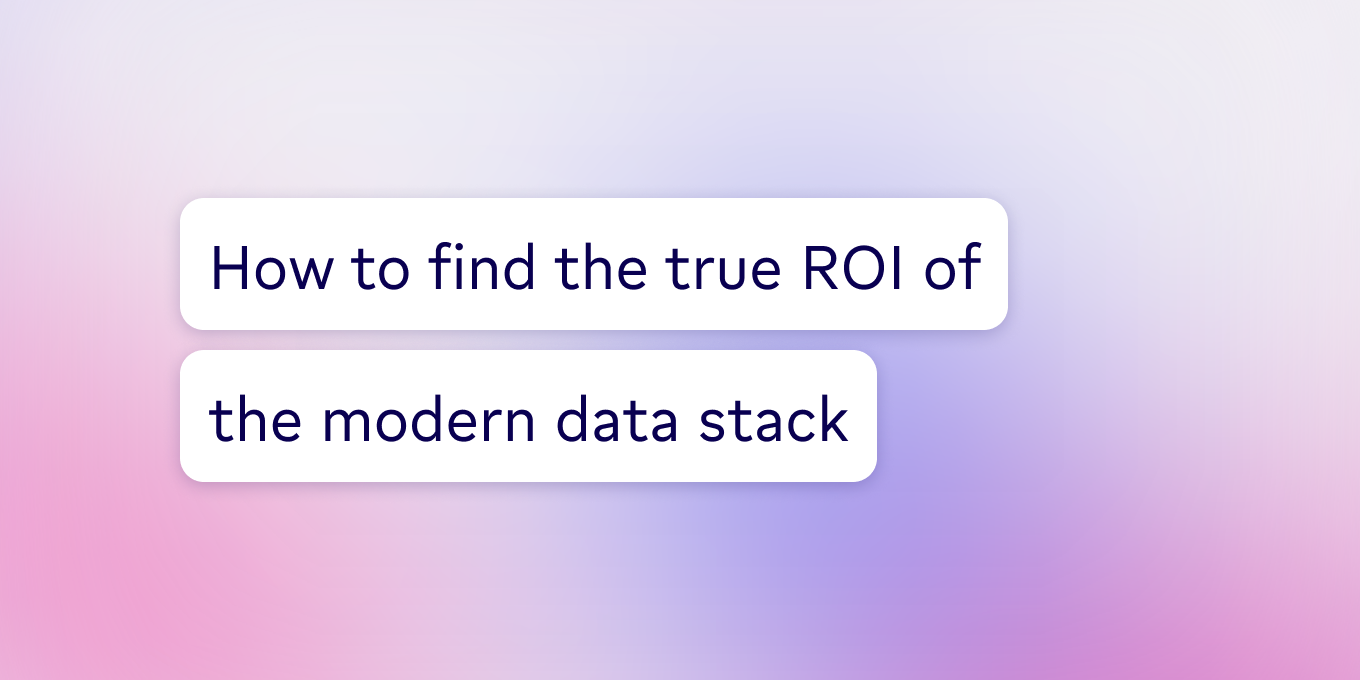
With more data flowing through companies than ever before, and competitors scrambling to be the best and fastest at operationalizing it, the modern data stack is increasingly seen as a must-have – at least among data folks.
But implementing these tools still requires buy-in from the business side. So, the burning question is, “How do we measure the ROI of the modern data stack?”
This was the main topic of discussion in our recent webinar, Proving the Value of Your Modern Data Stack with Google and Montreal Analytics. It featured some folks who do a lot of thinking about the impact your data stack has on ROI.
- Jobin George, staff solutions architect at Google
- Yoni Hazan, lead analytics consultant at Montreal Analytics
- Paul David, director of sales at Census
To kick things off, they all agreed: When making the case for data investment, you have to communicate the costs and value of both the tools and the people who run them. ⚒️➕😀
ROI = Goals achieved ✅
“It starts with the capital expenses – moving into a modern data stack or modernizing the tools you have,” said Jobin George, staff solutions architect at Google. “Once the migration is done, then comes the operating expenses. Who is going to run the show?”
Many stacks are a blend of first-party services, cloud providers, and third-party tools. Cost calculations should include the cost of the tool, consultants who help with migration, and ongoing use and support. With all those moving parts, it’s tricky to figure out where to attribute value.
“It’s difficult to pinpoint what is good across all companies,” agreed Yoni Hazan, lead analytics consultant at Montreal Analytics. “Tooling and personnel are line items that impact the finances of companies really differently. A small company and a large company are going to have different needs and require different skill sets.”
What you need from your data stack is likely to grow over time. You’ll eventually need more computation, more headcount, and more consultants.
But that might seem alarming to finance folks. 😱 To ease their anxieties, they have to see the return the company can expect from its modern data stack investment.
“Costs can be directly proportional to how much your team wants to achieve,” Jobin said. “Thoroughly research what your deliverables are so you don’t implement things you don’t really need. First, be sure you understand the problem, then invest only in the necessary areas to solve it.”
Beware of falling down the rabbit hole of marketing hype and buying tools that solve problems you don’t have. Because once you have the tool, you have to invent “problems” for it to solve just so you can justify the expense – and that’s a waste of time and money.
![]() |
Ultimately, choosing which problems to tackle all ties back to company goals. Data teams tend to be pretty good at setting objectives for themselves, but often forget to set goals that will move the entire business forward. ⏩
The elements of your modern data stack should directly connect to business objectives. So, if your company is really focused on reducing costs, you might turn up the heat on improving processes and automation. If you’re focused on revenue growth, you should concentrate on embedded analytics, data shares, and data clean rooms.
But even once all objectives are aligned and data folks take on more of a consultant role in their own organizations, they often struggle to connect business team results back to the data team’s contributions.
“The data team takes the costs and experiences few of the benefits,” said Paul David, director of sales at Census. “You don’t see sales teams proving the data team’s ROI by saying, ‘Hey, the data made this measurable impact in ROAS.’”
One way to combat this is to borrow the agile approach from engineering: You tackle specific, small goals to show value on a simple cadence. This framework is scalable, measurable, and helps stakeholders see the ROI. 💡
“It helps people understand what we’re trying to achieve and the value,” Yoni said. “What’s beautiful about the modern data stack is, within clicks, you can start generating value.”
Getting buy-in for the modern data stack
The biggest obstacle a lot of data teams face when making the case for modern data stack investment is sunk costs. Especially in a tough economy, it’s hard to convince the C-suite to fund a shift away from the systems and processes they’ve already invested in – even if everyone knows it’s the right move in the long run.
![]() |
Many legacy data stacks are built on "value” versions of open-source software self-supported by a team of smart data engineers. Jobin said he’s seeing a lot of companies migrating these stacks into fully-managed or semi-managed versions that let the data team hand off day-to-day management and focus on generating revenue.
“The call is between employing a bunch of really smart people on a mediocre platform or a small team of smart people on an awesome platform,” he said. “You have to choose the option that’s right for you.”
Investing in more managed systems can create stability in the entire data ecosystem. Paul said he’s seen plenty of instances where companies build processes that are cheap from a software perspective, but rely on one or two superhero engineers who wrote the custom script that runs everything. 🦸🦸♀️
“From a cost perspective, it’s particularly expensive to invest in getting a stack up and running when a single person leaving their role can bring the whole thing crashing down,” he said.
And when it comes to investing in tooling, ROI is often calculated on time to value. But since the value of the modern data stack relies on both tooling and personnel, companies also need to factor in onboarding time. That starts with the simple question, How long will it take a reasonably talented new hire to learn all the tools and processes so they can function as well as the rest of the team?
Making a case for a modern data stack means you have to frame the conversation so it doesn’t begin and end with the initial capital expense. Paint a picture of what can happen if you don’t modernize – if your data heroes leave and there’s no one to fill the gap.
“The beauty of the modern data stack is you don’t have to depend on somebody for their specific skill set,” Yoni explained. “When you have something broader, the team is scalable, and that makes the business scalable. The majority of value comes from documented processes and enabling the data team.”
Easing into investments
Before you start pitching decision-makers on your dream data stack, make sure every element can pull its weight. Here are some of the panel’s top tips:
- Painstakingly research the company’s objectives and the data team deliverables required to achieve them.
- Focus on the tools that make those deliverables possible with the least amount of manual intervention from the data team.
- When talking to business units about their goals, make sure everyone has a shared understanding of what terms mean. Different definitions of things like “activated customer,” “segment,” or “real-time” can have far-reaching impacts on how the data team does its job.
As you add tools to your data stack, Jobin also recommends sticking to pay-as-you-go licenses or licenses that last no longer than a year so you can test out a tool’s effectiveness before making a big commitment. Start with one project, he said. If that goes well, slowly start onboarding other workloads onto the platform, making sure it lives up to its hype.
Check out the full webinar with Jobin, Yoni, and Paul, where they talk more in-depth about establishing the ROI of the modern data stack here. 👇
💰 Want to see how Census can help you maximize the ROI of your data stack? Book a demo with a product specialist.