Data Quality: Ensuring Accurate Information
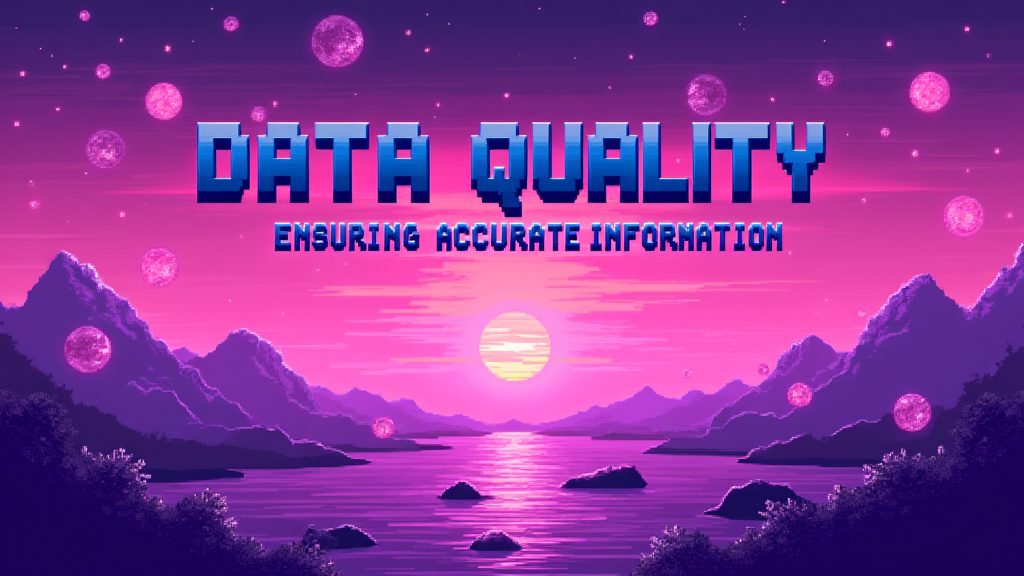
Published on: October 01, 2024
In the fast-paced world of Sales, Marketing, and Revenue Operations, data quality stands as a cornerstone for informed decision-making and operational efficiency. It refers to the overall reliability, accuracy, completeness, and consistency of data within an organization's systems and processes. 📊
Why Data Quality Matters in Operations
High-quality data is essential for:
- Accurate revenue forecasting
- Effective lead scoring and nurturing
- Personalized customer experiences
- Precise performance analytics
- Informed strategic planning
Poor data quality, on the other hand, can lead to misguided decisions, wasted resources, and missed opportunities. 😓
Dimensions of Data Quality
To truly understand data quality, it's important to consider its key dimensions:
Dimension | Description |
---|---|
Accuracy | Data correctly represents the real-world entity or event |
Completeness | All required data is present and recorded |
Consistency | Data is uniform across different systems and datasets |
Timeliness | Data is up-to-date and available when needed |
Validity | Data conforms to defined business rules and formats |
Implementing Data Quality Management
Maintaining high data quality requires a systematic approach:
- Data Profiling: Analyze existing data to understand its current state
- Data Cleansing: Correct or remove inaccurate, incomplete, or duplicate data
- Data Enrichment: Enhance data with additional relevant information
- Data Validation: Implement checks to ensure data meets quality standards
- Continuous Monitoring: Regularly assess and maintain data quality over time
The Impact of Data Quality on Revenue Operations
In Revenue Operations, high-quality data can significantly impact the bottom line. For instance:
- Accurate customer data can increase upsell opportunities by up to 20% 💰
- Clean data can reduce wasted marketing spend by 15-25% 📉
- Reliable sales data can improve forecast accuracy by 30% 📈
Common Data Quality Challenges
Organizations often face several hurdles in maintaining data quality:
- Data silos across departments
- Manual data entry errors
- Inconsistent data formatting
- Outdated or obsolete information
- Lack of standardized data governance policies
Tools and Technologies for Data Quality Management
Modern operations teams leverage various tools to ensure data quality:
- Data integration platforms
- Data cleansing software
- Master data management (MDM) solutions
- Data quality monitoring dashboards
- AI-powered data validation tools
Best Practices for Maintaining Data Quality
- Establish clear data quality standards and KPIs
- Implement automated data validation processes
- Provide regular data quality training to team members
- Conduct periodic data audits
- Foster a data-driven culture across the organization
By prioritizing data quality, Sales, Marketing, and Revenue Operations teams can unlock the full potential of their data assets, driving better decision-making and ultimately, improved business outcomes. 🚀
As you reflect on your organization's data quality practices, consider asking yourself:
- How confident are we in the accuracy of our current data?
- What processes do we have in place to maintain data quality?
- How can we improve our data quality to drive better business results?
- What tools or technologies could help us enhance our data quality management?