For the first 10 years of my career, the roles and team members in basic data warehousing projects remained static. First, there was the Business Analyst who would spend the majority of their time supporting the business with basic reports and simple analytics. Their computer desktop would be littered with a series of Excel documents containing complex pivot tables and VLOOKUPs to other spreadsheets. They possessed an intimate understanding of how the business operated and what metrics were required to create insights, forecasts and projections that would allow leadership to help make better business decisions.
The second was the Business Intelligence Developer role. As data volumes increased and data requests from the analyst became more formalized, Business Intelligence Developers would be responsible for building daily or nightly extract, transform and load processes to pull data automatically and store it in highly structured data warehouses with metrics, calculations, and mappings built right into the code. They created canned reports to replace some of the more tedious Excel-based reporting defined by the Business Analysts and broke down the data silos of an organization, ultimately giving the business a single source of the truth.
From a technology stack perspective, ETL tools such as SQL Server Integration Services, Informatica or even raw code would be used to create source to target mapping logic to load data into data warehouses, usually built on database technologies such as Oracle, Teradata, SQL Server or DB2 instances. Tools such as Cognos or SQL Server Reporting Services would then provide enterprise-wide reporting.
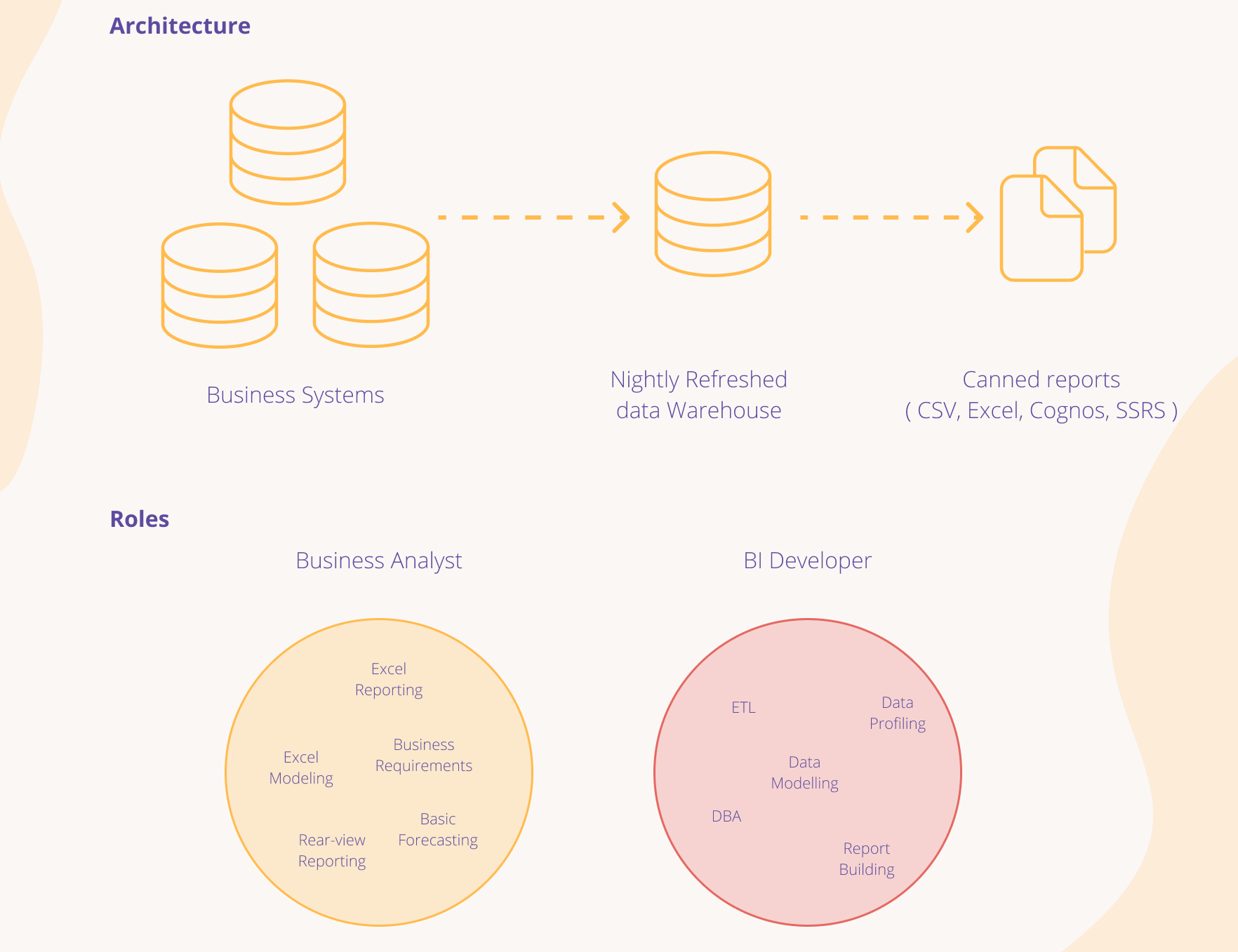
In the last 5-7 years, however, the business intelligence landscape began to shift as the big data revolution came about. With storage cost shrinking and large cloud-hosted data centers becoming the norm, the amount of data an organization can capture has grown exponentially by leveraging tools and services such as AWS Redshift, GCP BigQuery or Snowflake.
An Explosion of data
Data analysis exploded from the millions of rows to the billions or trillions range. As this shift happened, business intelligence tools also moved to the cloud which created products such as Looker, Mode, and PowerBI. They have specifically been designed to handle this new volume of data and offer new or expanded analytics capabilities such as:
- Ad Hoc and Self Service Reporting
- Slice and Dice
- Collaboration and Sharing
- Live and In-memory Data Analysis
- Integration of a variety of data sources
- Advanced Visualizations
- Maps
- Robust Security
- Mobile View.
- Natural Language Q & A Reporting
- Basic Modeling and Artificial Intelligence
Predictive Analytics evolved into Prescriptive Analytics. Nightly data refreshes can now be done in real-time, processing millions of rows every few seconds. Staging Databases have become massive infinite scaling data lakes. In addition, single server data warehouses have become multi-parallel processing data warehouses running on multiple clusters and nodes giving organizations the power to throw an infinite number of compute cycles at their queries and data processing.
More Data, More Responsibilities
As these changes to technology and capabilities came to life, the Business Analyst and the Business Intelligence Developer roles can no longer solely support all the needs being driven by this change. The Business Analyst role is now supplemented by a Data Scientist role.
The Data Scientists may not be as well versed in the intricacies of the business, but they bring Ph.D. level statistical analysis to the table allowing for in-depth complex analytics previously not possible by Business Analysts. Data scientists leveraged languages such as Python, R or SAS to create complex analytical models to drive the business forward.
The Business Intelligence Developer role has become generally more fragmented due to the various new steps in a Modern Data Stack. Although basic data warehousing principles are still required in this architecture, the data warehouse is just a single component rather than the sole home for all reporting and data. By having a robust and clean data lake, Business Analysts and Data Scientists no longer need to wait for a highly structured data warehouse to field reporting requests to answer problems requested by the C-suite with due dates of yesterday. The new role of Analytics Engineers has emerged as the leader in helping bridge the gap between Data Analysts and Data Scientists and the lightning-fast, exploratory analytics they need to create.
They help maintain flexible but clean data lakes while creating easy to process data pipelines to be leveraged by downstream data warehouses, automation, or real-time analytics. They have enough technical chops to understand concepts such as source control, software deployment life cycles and the other technical nuances of a software development project but also have enough business acumen to ensure the correct data points are being stored and categorized in all sections of the modern data stack so all teams that require the data have what they need to operate without confusion and with autonomy.
The Analytics Engineers are well versed in the swiss army knife of cloud-based tools. They are able to extract, transform, load, map and merge data from source data using tools such as dbt, SnowPipes, AWS Glue, Python to name a few. Data can then be loaded into leading edge MPP Data Warehouses such as AWS Redshift, Snowflake for structured and clean reporting.
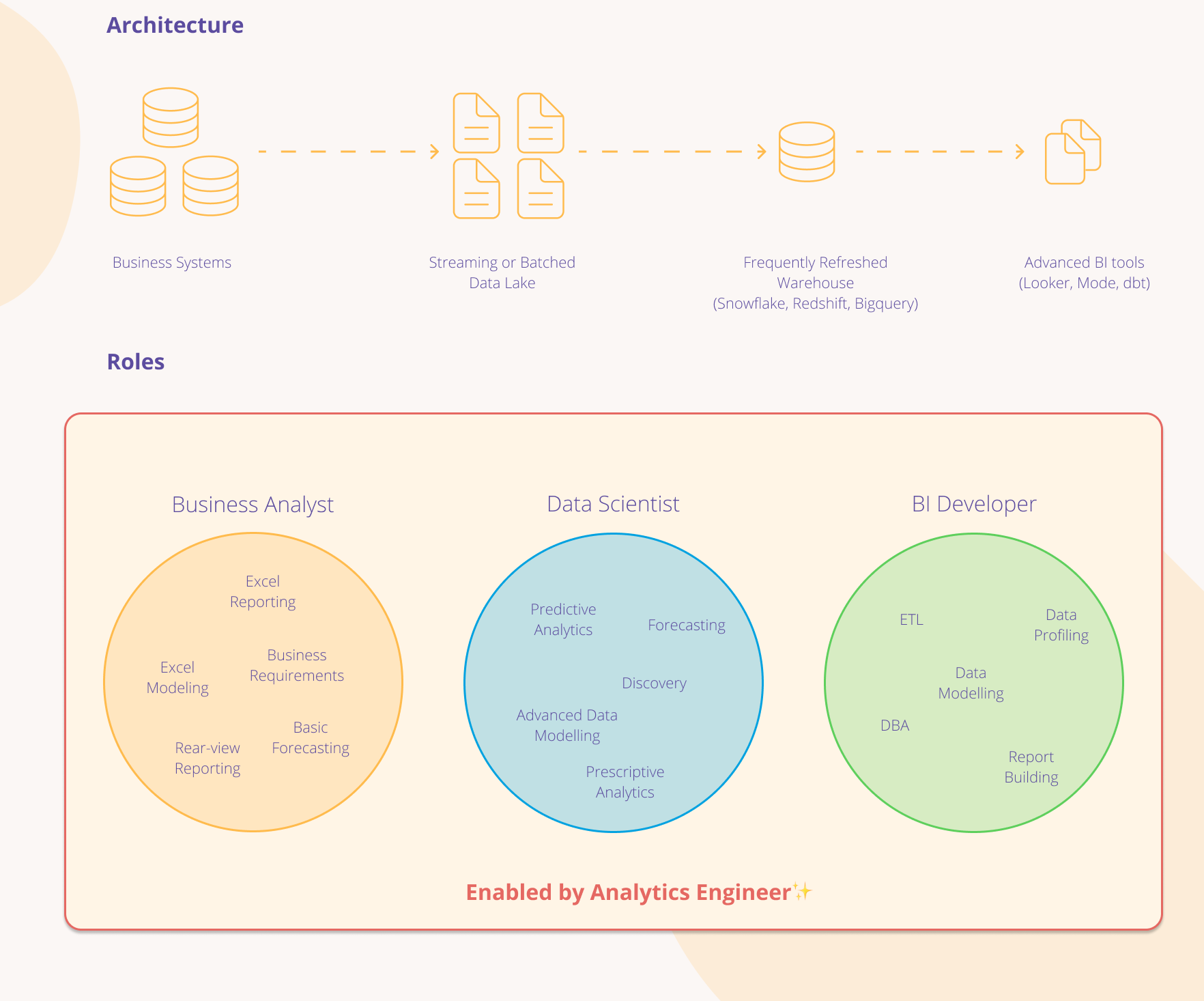
It may seem as allowing the Data Analysts and Data Scientists to connect directly to data lakes would eliminate the need for Analytics Engineers or the Business Intelligence Developers, but this has been found to create two major problems.
The first is that without proper care, data lakes have been known to become data swamps. Data is stored in mass volume, but without basic best practice architecture and engineering, the data is uncategorized, duplicated, stored incorrectly in addition to a myriad of other issues making the data unusable for Analysts and Scientists alike.
The other issue is that without an intimate understanding of the data, correct structures, and relationships, the recipients of the data run the risk of creating incorrect metrics that do not align to similar metrics being created by other business units to answer similar problems. The issue from the early data warehousing days of silo ghost IT departments with their own excel reports, reporting different numbers from adjacent business teams begins to reemerge. There is no longer a single source of truth achievable as everyone can run wild with an unorganized modern data stack that should be owned and facilitated by the Analytics Engineers.
What does the future hold?
Enabling the Analytics Engineers in-turn enables all other players in a well structured data organization. But an Analytics Engineers, like a good car mechanic, need the correct tools to do their job correctly. This is why you see more and more organizations adopting a modern data stack and leverage tools such as dbt, Fivetran, BetterException, etc the Analytics Engineer have all the power to do just that. If you are interested in building a better data organization or data stack, contact us, we do that for a living!