Here’s an activity for the data professionals reading this: Ask one of your stakeholders “What do you believe is the most efficient way for us to collaborate?” Odds are, your answer to this question and theirs may be misaligned.
Similarly, if a stakeholder were to ask you the same question, they’d likely be a bit surprised, too.
Sure, you could do this activity in any work relationship, and the reactions won’t be 100% satisfactory. However, I find the relationship between data professionals and stakeholders to be particularly interesting. As a result of the modern data stack era, data professionals have quickly evolved from a mere luxury to a necessity in organizations. Beyond simply building dashboards and helping inform long-term decision making, data professionals have inched closer to their organization's operations, and are tasked to support immediate revenue-driving activities. Not coincidentally, stakeholders are collaborating with data professionals more frequently than years prior.
Although collaboration has quickly become more frequent, it hasn’t improved at the same rate. I’ve observed this both personally and through various conversations with data professionals.
I’ve thought about this so much, in fact, that I decided to write a blog about collaboration in the modern stack world. This is not a straight-forward “how to” blog (So I guess the title is misleading!). I don’t believe organizations can simply adopt a new process to answer this question. Sure, processes make a significant impact, and we’ll go over them in detail, but education and a change in behavior is required from both parties, and that isn’t quite as easy as swiping a card and giving everybody a seat license.
First, let’s learn more about data professionals in the modern data stack world.
Data professionals in the modern data stack world
Rewind the clock 10 years and think about the responsibilities of data professionals. We were aggregating and cleaning data, generating reports, performing analysis, and providing insights. Our contributions were valuable, but the amount of data we collected was miniscule compared to today, and we weren’t as essential to an organization’s operations. Additionally, unless you suffer from the Mandela effect, the term “modern data stack” didn’t exist back then. Fast forward to today. In addition to the responsibilities above, we have a plethora of other responsibilities which piggy back off the modern data stack: automating “real time” workflows and pipelines, managing and maximizing each department’s frontline tools, enabling self-serve data access, etc. etc. As a result, we’re more central to our organization’s operations and revenue driving activities.
This increase in responsibility is fortunate (it gives us a seat at the table) but it also means we must develop a deeper understanding of our organizations as a whole and the OKRs of individual stakeholders. We should be more attentive and engaged in company-wide meetings and updates, goal planning sessions, etc. Through education, our deliverables will become more clear, the data we manage will become more purpose-driven, and we’ll collaborate more efficiently with stakeholders.
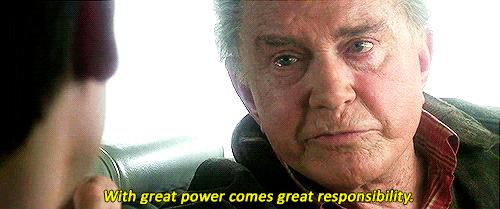
On the flip side, stakeholders should become more knowledgeable about data-driven culture, the modern data stack, and how it increases our responsibilities. It will help them better understand our bandwidth, capabilities, implications of requests, and ultimately help us collaborate more efficiently. If they fail to do so, trust will diminish. They’ll be disappointed in the outcomes and timelines of deliverables, and they’ll be less likely to achieve their OKRs.
Stakeholders in the modern data stack world
The modern data stack has made stakeholders (and any non-engineer employees for that matter) more data literate and technical, so much so that there’s even a term for these unofficial technical data users: citizen data scientists. Even in the absence of a top-notch data product, they constantly run their own analysis for short term decision making.
Just as the push toward PLG in recent years increased the attention given to data teams, it pushed stakeholders (and the ops teams below them) to learn more about customer data, and what that data means for their projects, campaigns, and targets. And with this more data-driven focus has come more data-driven OKRs, which (ideally) all stakeholder work is closely tied to.
This means, whether a stakeholder is in sales, marketing, customer success, etc. they utilize data and SaaS platforms to fulfill their responsibilities. SQL-savvy stakeholders frequently have a deep understanding of their tools, as well as a growing understanding of the tools in the data team’s arsenal, and they are increasingly capable of completing more than a handful of the requests they give to data teams.
So what does this mean for the data professionals? Well, it means that, much of the time, our stakeholders are the ones who understand what data they need best, because that data is directly related to the OKRs tied to larger business outcomes. As such, it’s pivotal that data teams recognize not only the growing technical expertise of the people requesting work from them, but honor that they know what data is most useful to them.
When they submit a simple request, or introduce a new, long-term data initiative, it is based on what they believe to be possible and manageable. I like how Sarah Krasnik described it:“It’s not the place for the [data] team to decide what data gets sent. You’re not the ones using the output, so you shouldn’t be the ones deciding the input.”If data professionals fail to understand their stakeholders’ needs and expertise, and fail to execute their requests, they won’t benefit their organization, and they’ll lose the trust of a key business ally. On the bright side, when both parties better understand each other's responsibilities and expertise, and change their behavior accordingly, the collaboration will become dramatically more efficient. Additionally, trust will grow between both parties rather than the typical ebbs and flows of disappointment. For example, a stakeholder is less likely to set an unrealistic timeline for a project when they have a baseline understanding of its requirements. Similarly, a data professional won’t throw a project on the backlog for several months when they recognize that a stakeholder’s request is directly tied to a major OKR.
How to collaborate more efficiently in the modern data stack world
As I mentioned earlier, this is not a straight-forward “how to” blog. The information below can help improve collaboration, but it will be in vain unless both parties understand each other’s responsibilities, and adapt accordingly.
I’ll focus on two different sectors to achieve efficient collaboration:
- Channels of collaboration. Where communications should happen depending on the problem at hand.
- How to collaborate. How to kick-off, plan, and manage a project. This is particularly useful for larger projects.
Channels of collaboration
Josh Richman (senior manager, business analytics, FLASH) and I discussed this recently in an OA coffee discussion. The topic was “How stakeholders and data professionals can collaborate more efficiently”, and it inspired me to write this blog! During the discussion, Josh shared the process that stakeholders use for requests:
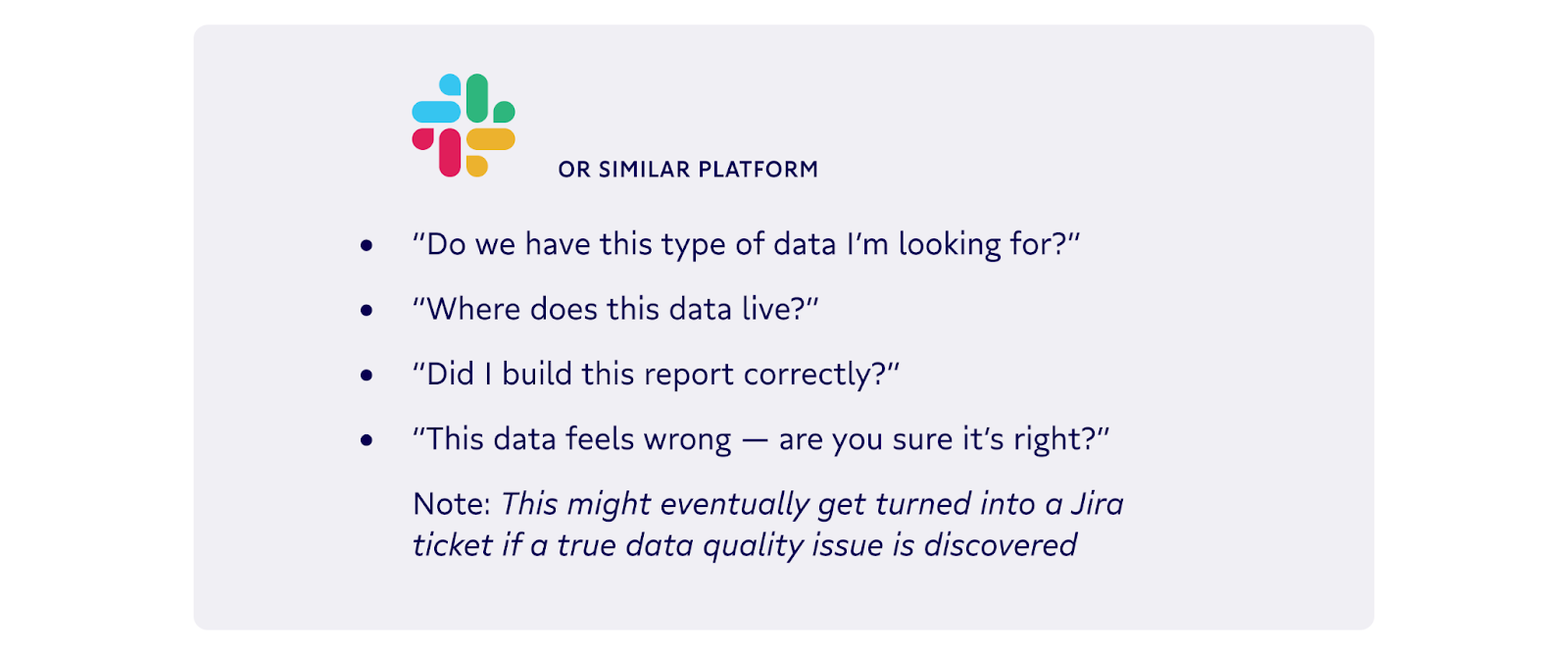
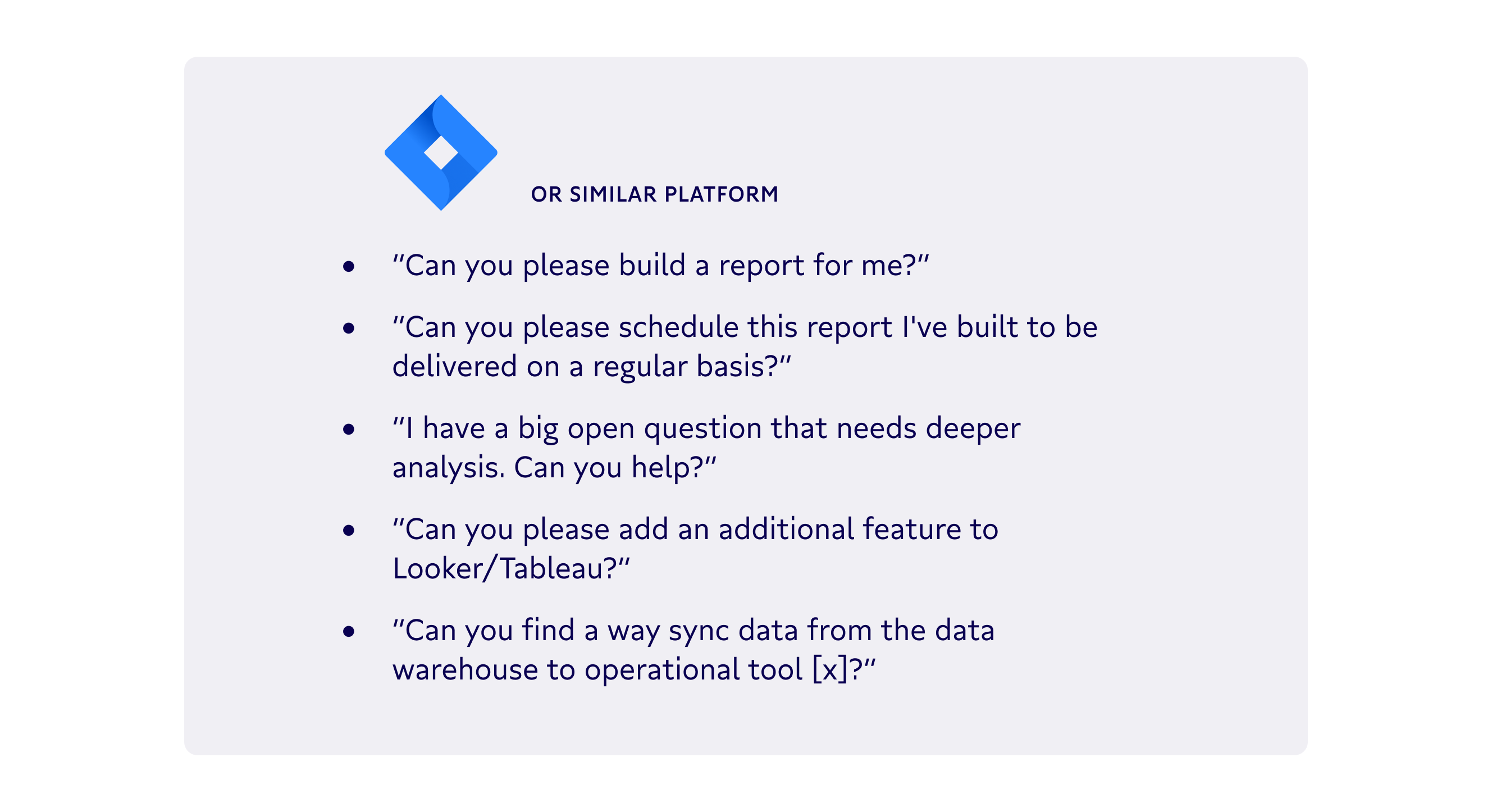
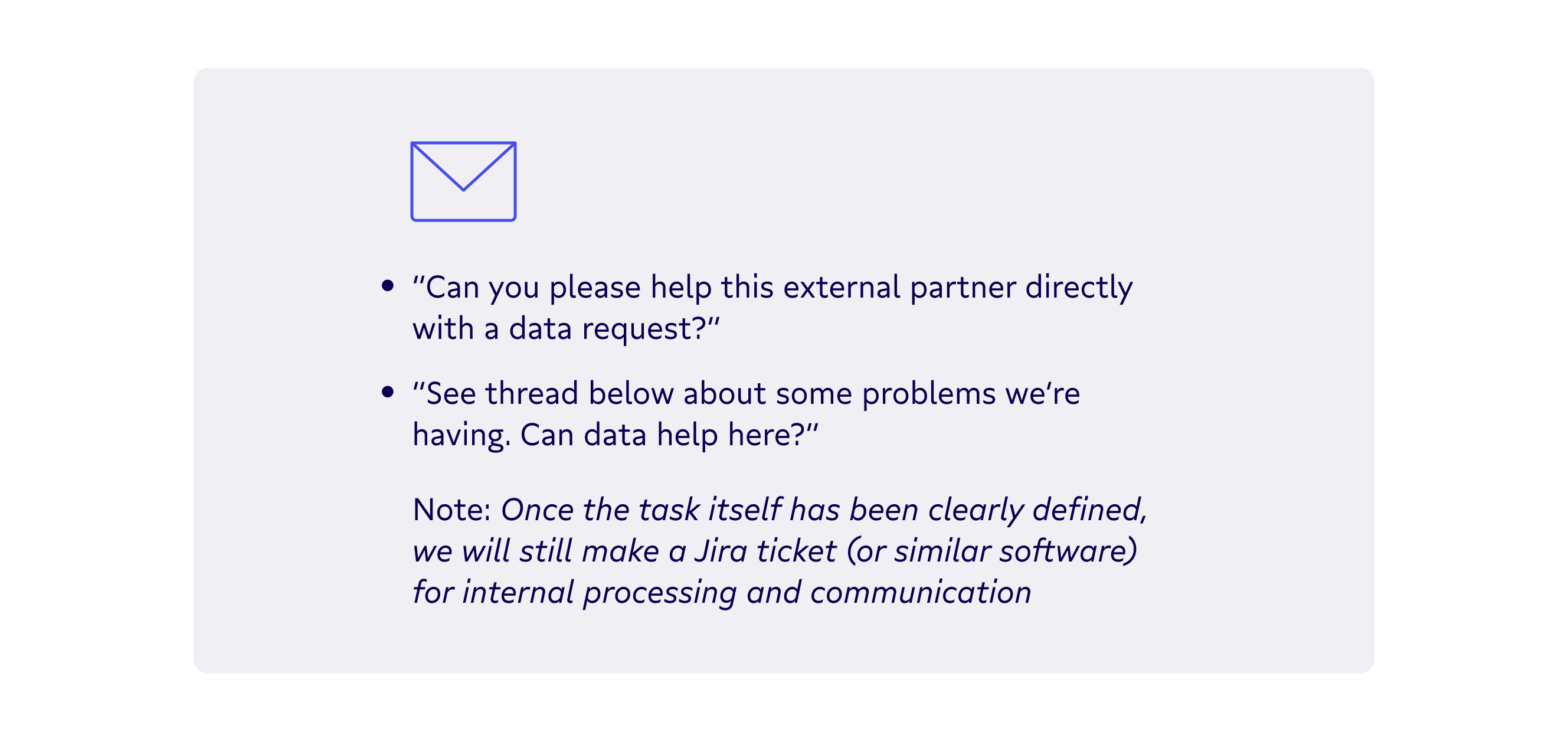
I like this mental model for thinking through collaboration methods because it keeps both parties organized and conscious of each other's time. Additionally, when larger requests live off the black hole that is Slack, they’re less likely to be forgotten about, and more likely to get completed in an appropriate amount of time.
Next time someone has a large, context-heavy request, perhaps ask them to fill out a Jira ticket or write an email accordingly.
How to collaborate
Irina Kukuyeva’s blog The "dark arts" of stakeholder management sums up how stakeholders and data professional collaboration better than I ever could. I highly recommend giving it a read.

In Irina’s 10+ years of stakeholder management, she’s found stakeholder alignment is more difficult than the actual analysis of data. You already have your own horror stories related to stakeholder misalignment, but you don't know that the outcomes can result in significant loss of trust and opportunity costs. To help avoid this, Irina put together a game plan. Here’s the TL;DR:
To ensure alignment for data projects (and collaboration in general), there are three questions that stakeholders and data professionals alike should aim to answer:
- What do you need to be on the same page? Context about problem, ideal outcome, deadlines, check-in cadence, etc.
- How do you know if you’re on the same page? Simply put, stakeholders are not surprised by the project plan or results!
- How do you actually try to get on the same page? Continuously check to make sure you’re providing all the needed context around the direction and progress of the work. Updates on blockers, managing additional requests, losing site of end goal, etc.
I/Irina believe that stakeholder management is more difficult than the actual data project. If you take away some of the best-practices she outlines, you’ll collaborate more efficiently with your stakeholders and deliver higher quality projects.
Where to go from here
I strongly encourage you to understand the responsibilities or your stakeholders and to make sure they understand yours. Be empathetic and remember: We’re all humans trying to make the best decisions with the data available to us. If a mutual understanding and respect isn’t present, moving to a new process will only make your collaboration improve slightly at best. Only if understanding and respect is mutual should you move forward with the “how to” collaboration suggestions in this blog. I hope it helps you collaborate better in this modern data stack world! If you have any questions, or if you’d like to discuss this topic more, you can find me (and the folks’ whose work I reference) in The OA Club, as well as via Twitter, LinkedIn, and email.