Lead scoring can help your team identify promising leads and intercept them with just the right amount of pressure and information to help them convert.
Traditionally, sales and marketing teams use lead scoring to assign a value or “score” to leads. These scores help teams identify people who might be open to sales calls or softer interactions within the customer journey. With the right systems in place, lead scores can trigger marketing automation or help sales teams predict the likelihood of closing a deal with a potential customer.
Lead scoring is a powerful resource to help you get more out of your lead generation efforts and find your highest converting channels. And the best part is: You don’t need the full modern data stack to start leveraging lead scoring today. You can use your existing marketing and sales systems to get going.
In this post, we’ll cover:
- Why is lead scoring important?
- How does lead scoring work?
- Getting started with lead scoring
- Data for lead scoring
- Lead scoring examples
- Tools to complement your lead scoring system
1. Why is lead scoring important?
Effective lead scoring accomplishes two things:
- It allows your teams to focus on the most engaged users.
- It helps them convert those users at a higher rate.
As your business scales, it becomes inefficient for members of your sales team to manually review each lead to determine whether or not they should move through the funnel. When as much as 70% of leads are lost from poor follow-up, you need to prioritize the time of your sales teams and give them the best leads.
"As a rule of thumb, the smaller the value of each sale compared to your total bottom line, the more important it is to process leads quickly, and the less it makes sense to dedicate an employee to understanding and assessing hard-to-quantify factors." - Boris Jabes, Census CEO
When your sales team can focus their time on your most highly qualified leads, your lead generation efforts yield higher results. Marketingsherpa reported that organizations using lead scoring experience, on average, a 77% increase in lead generation ROI compared to those that don’t.
2. How does lead scoring work?
Operationally, lead scoring uses a set of rules to “qualify” each lead in your pipeline, assigning it a new priority in your queue. The rules are based on which leads have historically converted to sales at the highest rates, which then informs predictive models to determine each lead’s score.
For example, if leads with Source = "referral" tend to convert more often than leads from other sources, you can use this information to prioritize following up on new leads that come from referrals. These rules become decision trees models use to rank your leads.
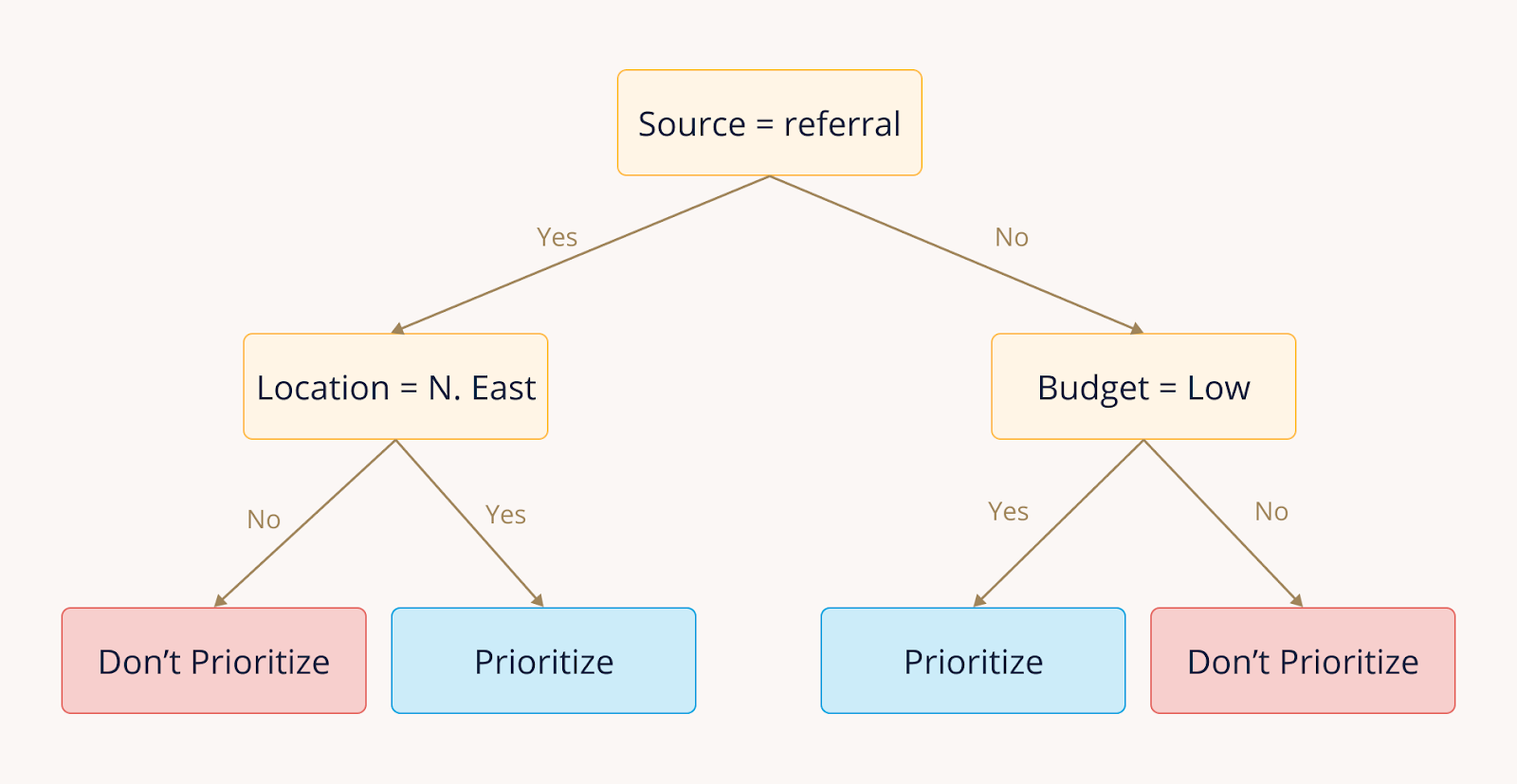
Types of qualified leads:
There are three main types of qualified leads between marketing, sales, and product.
- Marketing Qualified Leads: Potential customers who have interacted with your marketing efforts. They've read your blog, joined your mailing list, or downloaded your ebook. You can consider them actively curious.
- Sales Qualified Leads: Typically leads are handed off to sales that have somehow indicated they're considering making a purchase. You can label these as "hot leads."
- Product Qualified Leads: For teams building digital products or SaaS businesses, PQLs are potential customers who have already had a positive interaction with your product through a freemium plan or trial period.
Each type of qualified lead has achieved a specific set of qualifying actions, designed by your team to identify users who are most likely to become customers.
3. Getting started with lead scoring
A basic lead scoring system requires that you have a data stack in place, but it doesn’t have to be the complete modern data stack (though you’ll maximize your data even more as you build out the nervous system of your data at scale).
An example MVP of your data stack looks like this:
- Collection using a platform like Snowplow to gather customer data and send it to your warehouse
- Data storage in a warehouse like Snowflake
- A modeling tool like dbt to make your data usable in different situations
- Data activation tools like Census to load data into internal platforms
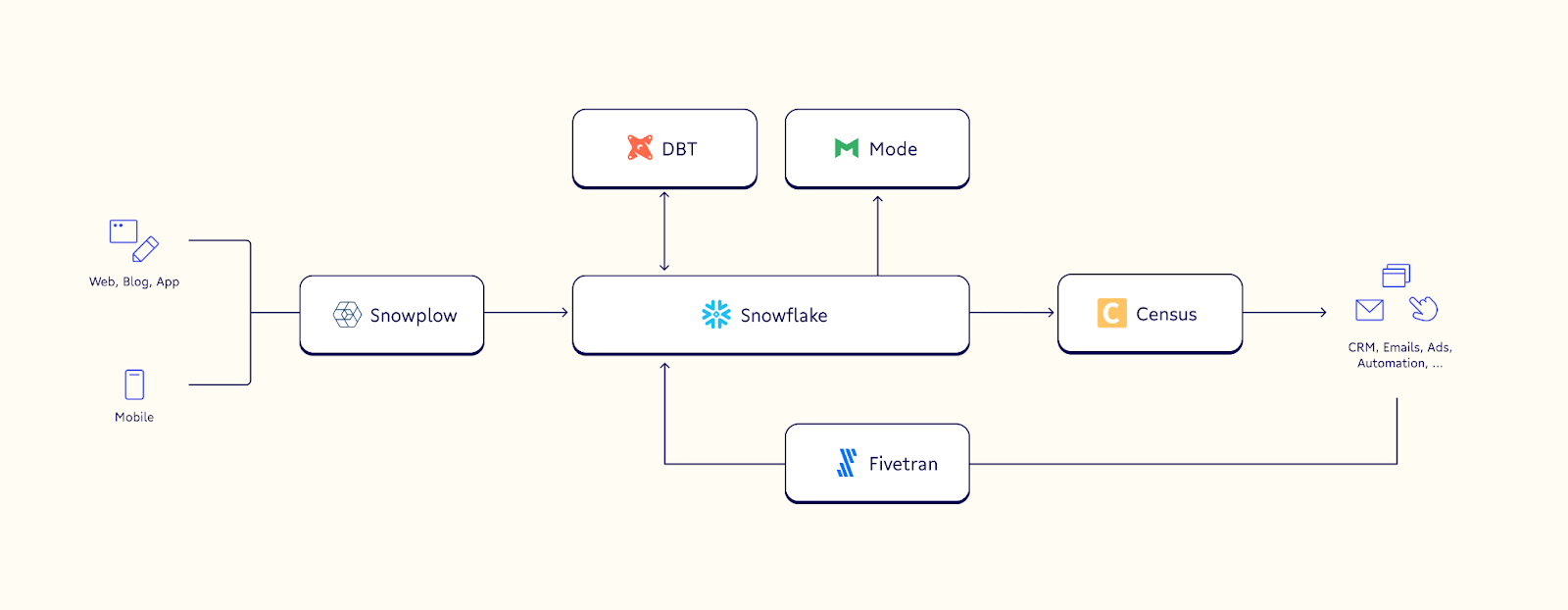
You’ll need a data collection platform to gather the information needed to fuel your lead scoring model. This data from each source gives you a more complete view of where your users are in their customer journey and makes your scoring more effective. Once you’ve collected the data, you need to load it into a warehouse where you can store and model it for future use cases.
Modeling tools like dbt give you access to large libraries of different data models, many of which you can use right out of the box for all but the most sophisticated use cases. You should tailor your predictive model to your business and customers, but generally, you can include points like a lead’s geographic region, associated company, previous web behavior, and historical email engagement across the board.
Once you’ve modeled your data, you can begin to empower your teams throughout the organization with rich data. The predictive model powering your lead scoring system can only inform your marketing automation or prioritize sales leads if those tools can access the data itself. Census, for example, pulls your usable data out of your warehouse, validates it, and sends it to your other internal platforms, ready to use.
4. Data for lead scoring
You know the saying, garbage in, garbage out. Your lead scoring model is only as effective as the data it ingests. As anyone who works with data can tell you, the most challenging work is often preparing the raw data before it’s used by your model. Optimizing your data collection reduces the difficulty of preparing your data downstream (and many platforms, including Census, also offer built-in validation tools).
If you’re using a data stack like the one described above, modeling tools like dbt and reverse ETL tools like Census help reduce the technical overhead required to work with your customer data and make it usable by the systems powered by your lead scoring models.
At a high level there are five key features that make data good for lead scoring:
- Data is stored in a standardized way
- Data is regularly snapshotted
- Leads can be uniquely matched to conversions
- You can identify currently active leads
- Data on historical lead prioritization exists
If you want to dive deeper into these features, check out our previous post on using machine learning for lead scoring.
Making sure you feed your lead scoring model quality data can be the difference between successfully prioritizing leads and sending your sales team down dead ends. When you get lead scoring right, you can see nearly a 200% increase in the average quality of your leads.
5. Lead scoring examples
For most teams, the lowest-lift, greatest-return for lead scoring models tend to be marketing email automation and PQLs. Let’s take a look at each.
Marketing email automation
Automating marketing emails allow you to build sophisticated lead nurturing campaigns or send personalized emails based on a lead’s engagement history.
A lead scoring model powered by data from each of your different sources can automatically trigger specific email campaigns with content customized for your users. Using the Census and Marketo integration, for example, you can make sure that any leads who read one of your blog posts are automatically notified when the next post in the series is published.
Product qualified leads
As mentioned above, PQLs are users that already have some experience with your product. They’re your freemium users or those whose demo periods have expired. Since they’re already fans of your product (🤞), PQLs convert at a higher rate and are less likely to churn once they become customers.
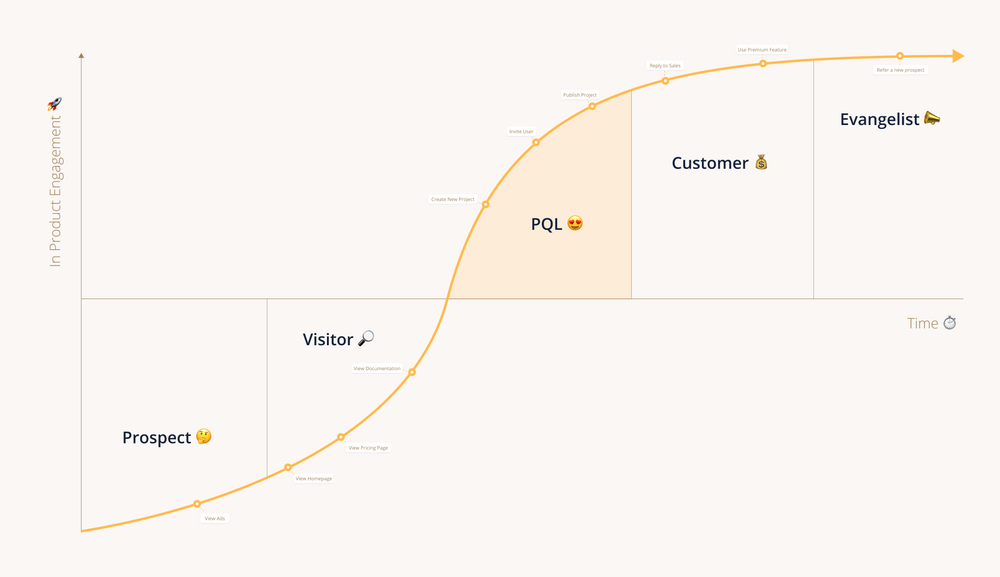
Your objective is to identify an action or series of actions that frequently lead to a free user becoming a paying customer. These actions become the rules that qualify leads as PQLs. If you know that a user who completes five specific actions is likely to convert, you can use your PQL model to identify users who have completed the first four and encourage them to complete the fifth.
6. Tools to complement your lead scoring system
A good lead scoring system gives you insight into what’s most important to your customers. This helps you reach your customers with the right type of engagement when it will have the greatest impact, improving their experience and your conversion rate. Effective scoring lets your sales and marketing teams focus on the leads most likely to become customers, saving them valuable time.
Building a lead scoring system requires you have some form of operational analytics stack. We recommend starting with the following.
- A way to collect and gather customer data
- A data warehouse
- A modeling tool to help use your data (optional, but very helpful)
- A way to share your modeled data with your teams
While the specifics of how you connect each piece of your stack will vary based on your unique set of tools, fundamentally the process is the same. With these pieces in place, you can begin building your lead scoring system. 🚀
As I’ve said, the stack above is a great MVP to get started with lead scoring, but if you’re missing a few pieces (or have a few extras you don’t know how to tie together) contact the Census team for a demo and they’ll help meet you where your data is today.