The value of data isn’t in the quantity you have, it’s in its usefulness. A huge dataset might make you feel like you have all the answers, but unless you can put it in context, all you have is a lot of noise. 📺
Take the weather, for example. While Census Co-founder and CEO Boris Jabes was chatting with Avo Co-founder and CEO Stefania Olafsdottir about the weather in her native country of Iceland, they broached the subject of wind. Despite how notoriously windy the country is, Icelandic weather forecasts rarely include comments about the wind – even though it can be the deciding factor between basking poolside or staying indoors.
“It’s almost like cultural context, which is hard to teach a computer,” Boris said. “I remember when I was a kid not understanding why there were all these supercomputers just for the weather. Now I understand: It’s because there are so many variables.”
While you might feel pretty confident in your decision to go to the pool based on comprehensive data about temperature, humidity, and precipitation, the wind helps put everything else into context. 🍃
Context is king 👑
In simple, data terms, context refers to the network of connections among data points. It affects everything in data collection – from what data points should be gathered to who gathers that data and, ultimately, how they define it. For that reason, Stefania is not a fan of the term “data scientist” because it can be used to describe so many facets of what data people do. Essentially, it’s an umbrella term that groups all data folks together without really labeling any of them properly. ☂️
“It’s probably similar to being a software engineer: You don’t hire generic,” she explained. “You can have 10 years of really juicy infrastructure engineering, but no skills whatsoever in front-end development. Data science is a similar thing.”
Data teams at most young companies start out just getting the leadership team spun up on and oriented to its inner workings; basically, providing a high-level rundown of where they are and what’s recently happened. A strong data analyst will eventually begin adding context to that data – so their reports are no longer looking backward, but looking forward. ⏩
The way they go from reporting on what happened last quarter to influencing the company's future direction is similar to how we add context to the data: By clarifying what we’re being asked to uncover. Every person on a data team should be challenged to respond to questions by asking, “What do you mean?” 🤔 Before diving into the “how”, data teams need to understand the “what” and “why.”
“There are so many layers to defining a question,” Boris explained. “There’s the dictionary definition of the word, there's the semantics within our company, and there’s the final version they actually want you to answer.”
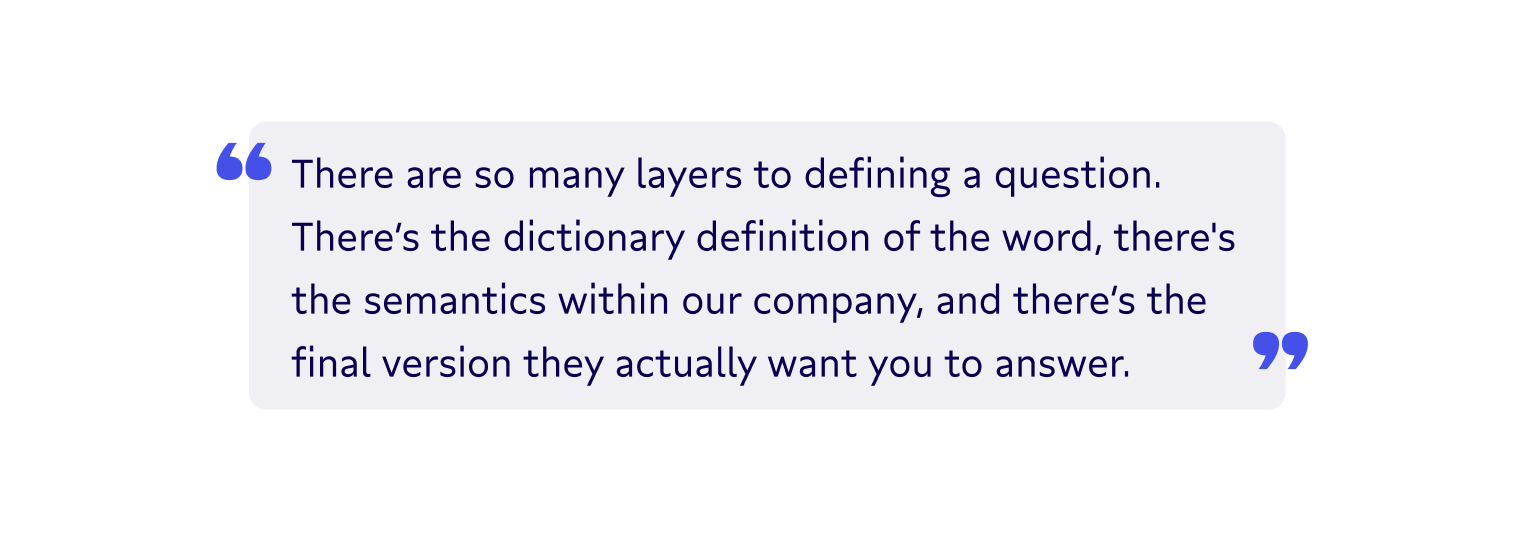
Take a straightforward question like, how many users do we have? Before you can answer that question, you need to understand how “user” is being defined.
Do you become a user when you download the app?
When you accept the terms and conditions?
Or not until you’ve completed an activity within the app?
We’ve said it before, and we’ll say it again: Context 👏 is 👏 everything. 👏
When you know the contextual parameters of a question, you can build an infrastructure that is strategically designed to evolve as the pertinent questions mature.
Inevitably, definitions will shift over time. Metrics and KPIs will change as products move through their lifecycles. A forward-looking data team will be aware of whether they want to answer different versions of the question in the future, or if the data is structured to provide only one definition. Is the infrastructure evolutionally intelligent?
“You need the flexibility to modify how you gather data while staying consistent, so you can report on things year-over-year,” Stefania said. “But at the end of the day, you have to start somewhere. Don’t try to make it perfect – because it will never be perfect.”
A shared frame of reference aligns teams
Contextualizing your data makes it less abstract to other teams in your company. When your data makes more sense and your data team can internally show how its findings impact business decisions, you can get your product and development teams amped up about analytics. 💪
Not only does this hype make product and development folks excited to include the data team earlier in the development cycle, but it also reduces the once-wasted time and resources associated with including the data team at the very end of the development cycle. When you incorporate the data team at the start of your development cycles, you can streamline the decision-making process, driving strategic decisions earlier.
“It’s a company milestone to have the product and development teams excited to understand the impact of what they’re doing,” Stefania said. “Such a huge part of how metrics are used involves having stakeholders aligned on what you’re trying to measure. That turns into the product stakeholder, the data stakeholder, the developer, and the designer talking about the goal of the feature and how to measure its success before it’s released.”
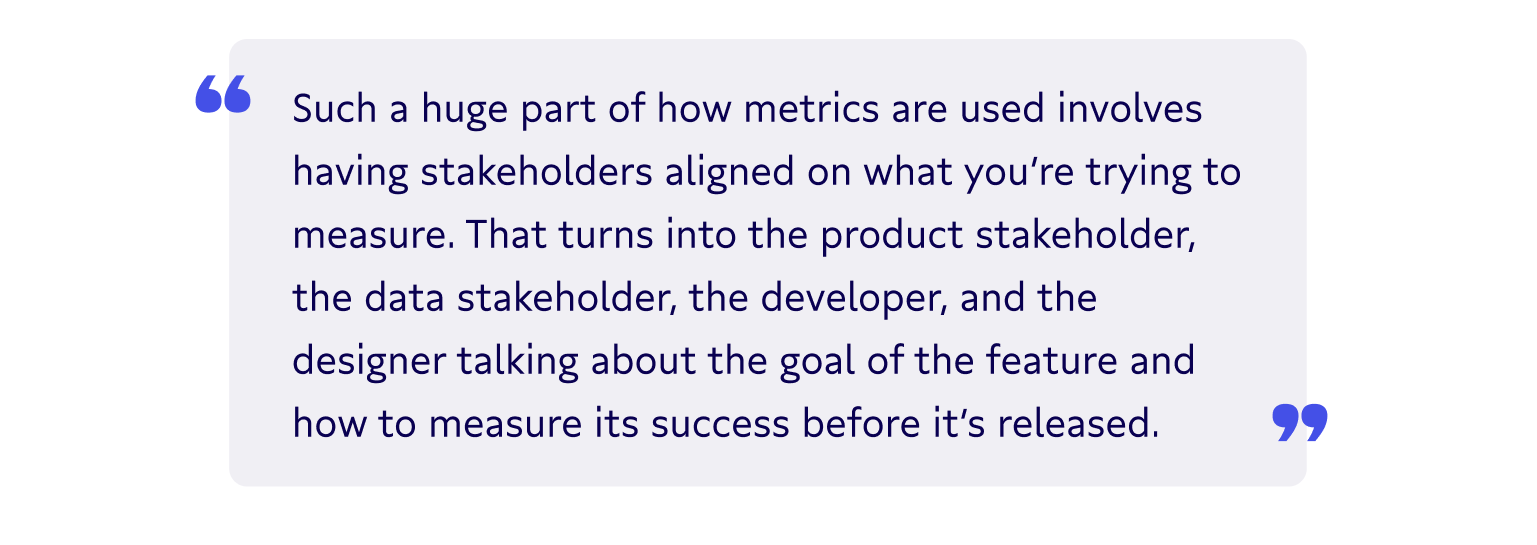
In a positive product culture, the data team has minimal involvement in developing the product roadmap, but even if they're uninvolved in the roadmap creation, they're still included in the conversation from the very beginning.
Why? Those conversations provide the crucial context that helps the data team think about what questions need to be answered to deliver the insights the company needs.
So, let's backtrack to the wind discussion. A simple question like, “How warm will it be on Friday?” could be answered with “70 degrees.” That sounds perfect without context, but with prior context, the data team can know what you’re really wondering is, “Will Friday be a good day to schedule a picnic?”
Now, if that 70-degree temperature forecast is predicted to be paired with 40 mph wind gusts, that likely changes the answer to, “Friday might not be the best day for a picnic.” 🥶
A single data point is useless. As a matter of fact, even hundreds or thousands of data points are useless on their own. The truth is, no matter how rich your data is or how diverse your data sources are, context is key to delivering real, measurable impact.
Want to learn more? You can catch the full conversation between Boris and Stefania below, or on your favorite streaming platforms. 🎧
Got thoughts and opinions about this topic? Join the conversation around this, and many other data best practices, in ✨ The Operational Analytics Club. ✨