You were deep in an analytical flow, firing off every question that you think you Snowflake DB can answer – and then, it happened. 😱 You got the unnecessarily terse and unexpectedly confusing error, “blah blah blah is not a valid group by expression.”
You might be too tired to catch an obvious problem, or it just might be time to level up your SQL skills with a lesson on Window functions. Let’s find out, shall we?
Invalid GROUP BY Expressions
A typical compilation error that any Snowflake user has run into is " … is not a valid group by expression." These errors are so common because you can run into them in two different ways. 👇
- By accidentally omitting or adding pieces of code (also known as "slips"),
- By writing incorrect code that you expected to work (also known as "mistakes").
Either way, when the error occurs, it always means that there's something in your query's GROUP BY clause that is not supposed to be there. The next section describes two examples (one slip & one mistake) that caused the error.
Two things can cause this error
Most likely, you’re here because you commented out a header column in your SELECT clause (but not in your GROUP BY clause) – or you’re still wrapping your head around Window functions entirely. 🤔
Either way, we're walking through two possible scenarios. The first cause we’ll walk through is a typical slip: Adding an aggregated column to a GROUP BY function. But the second cause is fairly complex: Referring to unaggregated columns in a Window function without using the columns in the GROUP BY clause.
In these code samples, we’ll follow a typical operational analytics use case: Rolling up customer orders onto customer accounts to sync to a CRM tool like Salesforce. For this, we’ll use data from Snowflake’s TPC-H benchmark data set.
Prerequisites
You probably have your own error you're dealing with, but we wanted to make this easy to play around with, so we made it easy to reproduce these errors. (You’re welcome?) 💁
The following code samples require – you guessed it – a Snowflake account with access to the TPC-H benchmark data set. All queries can be executed in Snowflake’s web interface via SnowSQL CLI or, if you really want to, the Snowflake API.
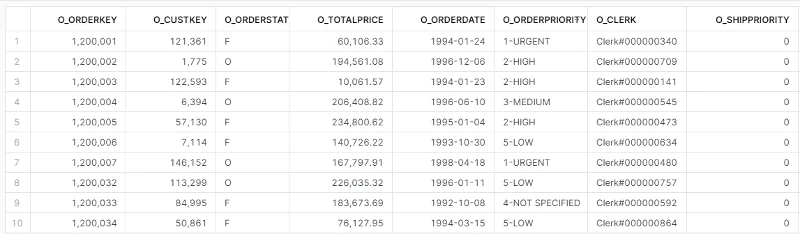
Let’s cover the simple error cause first, then get into the nitty-gritty.
Aggregated Columns in the GROUP BY Clause (aka oops!) 😬
If we want order summary metrics for each account, we’ll need to group each account’s orders together. As you know, a GROUP BY clause is used to group rows that have the same values into "summary rows." The code in the example below is trying to list the total value (the sum of O_TOTALPRICE) and the number of orders (a count of O_ORDERKEY) per customer (C_CUSTKEY).
SELECT c.c_custkey,
SUM(o.o_totalprice),
COUNT(o.o_orderkey)
FROM tpch_sf1.orders
GROUP BY 1,
2 -- this second mismatched column reference is the problem
However, an additional column has been introduced into the code so that it produces the compilation error "[SUM(O_TOTALPRICE)] is not a valid group by expression."
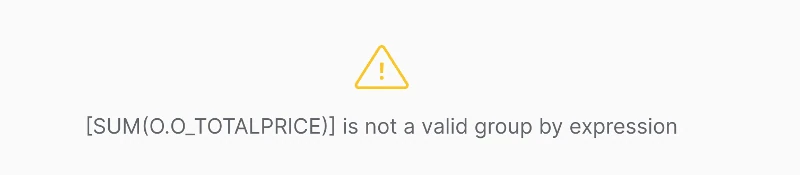
In this example, the error is produced because there's been an aggregation function added in the GROUP BY clause. The number 2 refers to the second column in the SELECT statement, which is the O_TOTALPRICE column wrapped in the SUM function. Because aggregations aren't allowed inside GROUP BY statements, Snowflake throws the error.
Why would anyone try to do this? The truth is that it will most likely happen unintentionally. 🤷 For example, you might run into it if you've removed a line in the SELECT clause. That's why it's recommended that you write the column names out in the GROUP BY clause; you'll be able to spot the slip a lot quicker.
Fixing it is easy; just match up the columns in your SELECT clause with the columns or shorthand numeric references in the GROUP BY clause! If that doesn’t fix it, you’re likely going to need to continue to the next section.
Unaggregated Columns in Window Functions
There's a more complex way to generate the "...is not a valid group by expression" compilation error using a window function.
Window functions are tough to define without an example, so I urge you to read this visual explanation if you're new to the subject. Snowflake's documentation on the topic comes down to the following sentence: A window function's "output depends on the individual row passed to the function, and the values of the other rows in the window passed to the function."
If you don’t understand that, it means you’re human. 😅 But don’t worry, its most important business use cases are rolling averages and running totals.
In the next example, we’ll combine Window functions with a GROUP BY clause, which, in many SQL dialects, simply won't work. Luckily, the Snowflake dialect supports the combination of both. However, the documentation page comes with a dire warning:
PARTITION BY is not always compatible with GROUP BY
The query below attempts to create a result set that shows the customer ID, the order status, and two calculations:
(1) the revenue per order status (REV_PER_CUSTKEY_ORDERSTATUS), per customer
(2) the revenue per customer ID, broadcast to all rows.
In the common analytical use of this query, your next step would be to divide (1) by (2) so that you get the revenue share per order status for each customer.
SELECT o_custkey,
o_orderstatus,
sum(o_totalprice) AS rev_per_custkey_orderstatus,
sum(o_totalprice) OVER (PARTITION BY o_custkey) AS rev_per_custkey
FROM tpch_sf1.orders
GROUP BY 1,
2
However, the query produces the following error: "[ORDERS.O_TOTALPRICE] is not a valid group by expression." So, what's going on here?
Your first clue of the issue at hand can be found by taking a look at this documentation page. It states the following: “Many window functions and aggregate functions have the same name. For example, there is a SUM() window function and a SUM() aggregate function.”
In the query above, our thought process expects the second SUM() function to aggregate O_TOTALPRICE. However, it is a Window function, not an aggregate function, and typically, a SQL query processes the GROUP BY clause before Window functions.
A SQL query that contains GROUP BY can produce columns that are either listed in the GROUP BY clause or wrapped in an aggregate function. Since the second SUM() is a Window function and not an aggregation function, Snowflake's compiler refuses to process the query.
You have two options to resolve this.
The first solution is to wrap an extra SUM() around the SUM() aggregation function. The nested SUM() is now an aggregation function, and the outer SUM() is the Window function. Now that you've introduced an aggregation function, the GROUP BY clause's requirements are met like so.
SELECT o_custkey,
o_orderstatus,
sum(o_totalprice) AS rev_per_custkey_orderstatus,
sum(sum(o_totalprice)) OVER (PARTITION BY o_custkey) AS rev_per_custkey
FROM tpch_sf1.orders
GROUP BY 1, 2
ORDER BY o_custkey,
o_orderstatus
However, if you think that nested SUM() functions look silly (you're not alone!), you can obtain the same result via a CTE. Sure, it makes your query a lot longer, but its readability improves drastically.
In the CTE, you calculate the sum of the revenue per customer ID and order status. The result is that you no longer need a GROUP BY clause in our main query and can use window functions without any problems. Check it out.
WITH first_cte
AS (
SELECT o_custkey,
o_orderstatus,
sum(o_totalprice) AS rev_per_custkey_orderstatus
FROM tpch_sf1.orders
GROUP BY 1,
2
)
SELECT o_custkey,
o_orderstatus,
rev_per_custkey_orderstatus,
sum(rev_per_custkey_orderstatus) OVER (PARTITION BY o_custkey) AS rev_per_custkey
FROM first_cte
ORDER BY o_custkey,
o_orderstatus
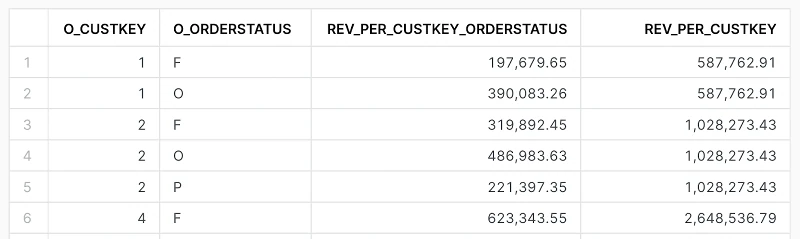
And that’s it! You get your cake, and you can eat it too! 🎂 (Or go ahead and substitute that with whatever culturally relevant expression is more appropriate for you—or your favorite food of choice.)
The more you know
This article explained what compilation errors are and how a "... is not a valid group by expression" is typically created. While it's easy to produce this error, there are some advanced use cases where it might appear unexpectedly, such as when combining a GROUP BY clause with Window functions. Luckily, these errors can be resolved in different ways.
📈 Want to up your SQL skills? Check out our free SQL workshop with Ergest Xheblati, author of Minimum Viable SQL Patterns. Check it out!