Master Data Management (MDM) is a critical discipline in the field of data management that enables organizations to centralize and maintain consistent, accurate, and high-quality master data.
By establishing a single source of truth for key entities such as customers, products, and vendors, MDM ensures data consistency and integrity across various systems and platforms. In this comprehensive guide, we will explore the concept of MDM and more.
1. Introduction to Master Data Management
Master Data Management (MDM) is a discipline aimed at managing and maintaining the master data of an organization. But what exactly is master data? Master data refers to the core entities that are crucial to the operations of a business, such as customers, products, employees, and vendors. These entities are commonly shared across multiple systems and applications within an organization. MDM focuses on creating a single, authoritative source of master data to ensure consistency, integrity, and accuracy.
Definition and Importance of MDM
Master Data Management can be defined as the process of creating, managing, and distributing master data across an organization to ensure data consistency, accuracy, and integrity. The goal of MDM is to provide a unified and accurate view of master data, eliminating data silos and inconsistencies that may arise from disparate systems and applications.
The importance of MDM in today's data-driven world cannot be overstated. Organizations generate vast amounts of data each day, and it is crucial to have a solid foundation of accurate and consistent master data. MDM enables businesses to make informed decisions, improve operational efficiency, enhance customer experiences, and comply with regulatory requirements.
Key Components of MDM
Master Data Management comprises several key components that work together to ensure the success of MDM initiatives. These components include:
- Data Governance: Data governance establishes policies, procedures, and responsibilities for managing and maintaining master data. It ensures that data is accurate, consistent, and compliant with regulations.
- Data Quality Management: Data quality management focuses on assessing, improving, and maintaining the quality of master data. It involves activities such as data profiling, cleansing, and standardization.
- Data Integration and Consolidation: Data integration and consolidation involve bringing together master data from various sources and systems into a centralized repository. This process eliminates data redundancies and inconsistencies.
- Data Security and Privacy: Data security and privacy are crucial aspects of MDM. It involves implementing measures to protect master data from unauthorized access, ensuring compliance with data protection regulations.
- Continuous Monitoring and Maintenance: MDM is an ongoing process that requires continuous monitoring and maintenance to ensure data accuracy and integrity. Regular audits, data cleansing, and updates are essential to keep the master data up-to-date.
Benefits of Implementing MDM
Implementing Master Data Management offers a wide range of benefits for organizations. Some of the key benefits include:
- Improved Data Quality: MDM ensures that master data is accurate, consistent, and reliable. This improves the quality of data used for decision-making and operational processes.
- Enhanced Operational Efficiency: By centralizing and standardizing master data, MDM eliminates data redundancies and inconsistencies, leading to improved operational efficiency and streamlined business processes.
- Better Business Insights: MDM provides a single, unified view of master data, enabling organizations to gain valuable insights into customers, products, and other key entities. This, in turn, supports data-driven decision-making.
- Compliance with Regulations: MDM helps organizations comply with data protection and privacy regulations by establishing data governance policies and implementing security measures to protect master data.
- Improved Customer Experience: With accurate and consistent customer data, organizations can provide personalized experiences, targeted marketing campaigns, and better customer service.
- Increased ROI: Implementing MDM can result in a positive return on investment, as it improves data quality, operational efficiency, and business insights.
In the next section, we will delve into real-life examples of successful MDM implementations and how organizations have benefited from adopting MDM strategies.
2. Examples of Successful Master Data Management
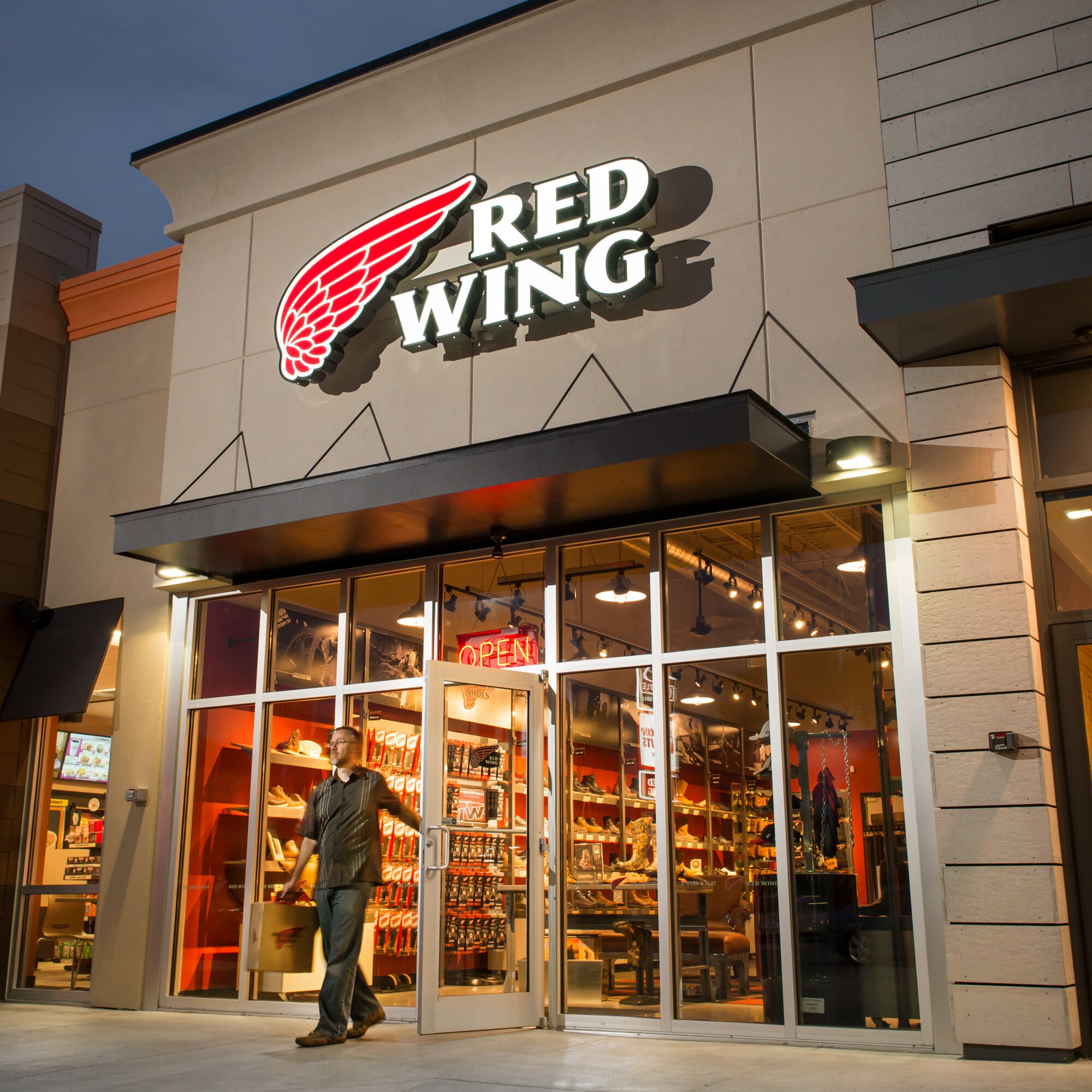
Red Wing Shoes: Consolidating Data for Operational Efficiency
Red Wing Shoes, a renowned footwear company, embarked on a journey to implement MDM to consolidate and streamline their data across various systems. By centralizing their customer, product, and vendor data, Red Wing Shoes achieved operational efficiency and improved data accuracy.
Prior to implementing MDM, Red Wing Shoes faced challenges in maintaining consistent customer information across their retail stores, e-commerce platform, and customer service centers. With MDM, they were able to create a single, unified view of customer data, enabling them to provide personalized experiences, targeted marketing campaigns, and improved customer service.
Not only did MDM improve customer experiences, but it also enhanced Red Wing Shoes' operational efficiency. With accurate and consistent product data, they were able to streamline their inventory management, pricing, and product catalog processes. This resulted in cost savings, reduced errors, and improved overall efficiency.
IBM: Unifying Customer Data for Personalization
IBM, a global technology company, implemented MDM to unify their customer data and enable personalized experiences for their clients. With a vast customer base and a wide range of products and services, IBM faced challenges in identifying and understanding their customers' needs and preferences.
By implementing MDM, IBM was able to consolidate customer data from various sources, including CRM systems, sales databases, and marketing platforms. This allowed them to create a comprehensive Customer 360 view, which provided a holistic understanding of each customer's interactions, preferences, and history with the company.
With the Customer 360 view, IBM was able to deliver personalized experiences, targeted marketing campaigns, and tailored product recommendations to their clients. This helped them build stronger customer relationships, enhance customer loyalty, and drive business growth.
Multinational Organization: Achieving Compliance through MDM
A multinational organization, implemented MDM to achieve compliance with data protection and privacy regulations, such as the General Data Protection Regulation (GDPR) and the California Consumer Privacy Act (CCPA).
Prior to implementing MDM, they struggled with data privacy and compliance issues, as they had multiple systems and databases that stored customer, employee, and supplier data. This fragmented data landscape made it challenging to ensure compliance with the ever-evolving data protection regulations.
With MDM, the organization established data governance policies, implemented security measures, and centralized their master data. This enabled them to manage and protect personal data more effectively, ensuring compliance with regulations and building trust with their customers and stakeholders.
In the next section, we will explore key strategies for achieving MDM success, including establishing a data governance framework and ensuring data quality management.
3. Strategies for Achieving MDM Success
Implementing Master Data Management requires careful planning and execution. To ensure MDM success, organizations should consider the following strategies:
Establishing a Data Governance Framework
Data governance is a critical component of MDM and provides the foundation for successful MDM implementation. It involves establishing policies, procedures, and responsibilities for managing and maintaining master data. A robust data governance framework ensures that data is accurate, consistent, and compliant with regulations.
Key elements of a data governance framework include:
- Data Governance Council: Establishing a cross-functional council responsible for setting data governance policies, resolving conflicts, and making strategic decisions related to master data.
- Data Stewardship: Assigning data stewards who are responsible for data quality, data integrity, and ensuring compliance with data governance policies.
- Data Standards and Policies: Defining data standards, data definitions, and data quality requirements to ensure consistency and accuracy of master data.
- Data Governance Processes: Implementing processes for data profiling, data cleansing, data integration, and data lifecycle management.
By establishing a robust data governance framework, organizations can effectively manage and maintain master data, ensuring its accuracy, consistency, and integrity.
Data Quality Management
Data quality is a crucial aspect of MDM. Poor data quality can lead to incorrect insights, inefficient operations, and poor decision-making. To achieve MDM success, organizations must prioritize data quality management.
Key steps in data quality management include:
- Data Profiling: Analyzing and evaluating the quality of master data to identify data anomalies, inconsistencies, and inaccuracies.
- Data Cleansing: Removing or correcting data anomalies, inconsistencies, and inaccuracies to improve data quality.
- Data Standardization: Applying standardization rules to ensure consistent data formats, values, and definitions across systems.
- Data Validation: Implementing validation rules to ensure that data entered or imported into the MDM system meets predefined criteria.
- Data Monitoring: Continuously monitoring data quality to identify and address data issues in a timely manner.
By implementing robust data quality management processes, organizations can ensure that their master data is accurate, consistent, and reliable.
Data Integration and Consolidation
Data integration and consolidation play a crucial role in MDM. They involve bringing together master data from various sources and systems into a centralized repository, often referred to as a master data hub. This eliminates data redundancies, improves data consistency, and provides a unified view of master data.
Key steps in data integration and consolidation include:
- Data Mapping: Mapping data elements from different source systems to the corresponding master data attributes in the MDM system.
- Data Transformation: Transforming data from different formats and structures to ensure compatibility and consistency within the MDM system.
- Data Loading: Loading transformed data into the MDM system, ensuring that it is accurate, complete, and up-to-date.
- Data Synchronization: Implementing processes to keep the master data in sync with the source systems, ensuring real-time updates and consistency.
By integrating and consolidating master data, organizations can eliminate data silos, improve data consistency, and provide a unified view of master data.
Data Security and Privacy
Data security and privacy are critical considerations in MDM. Organizations must implement measures to protect master data from unauthorized access, ensure data confidentiality, and comply with data protection regulations.
Key steps in ensuring data security and privacy in MDM include:
- Access Control: Implementing role-based access control to ensure that only authorized users have access to master data.
- Data Encryption: Encrypting sensitive master data to protect it from unauthorized access or breaches.
- Data Masking: Masking or anonymizing sensitive master data when it is used for development, testing, or analysis purposes.
- Data Privacy Policies: Establishing data privacy policies that outline how personal data is collected, processed, and stored, ensuring compliance with data protection regulations.
By implementing robust data security and privacy measures, organizations can protect master data from unauthorized access, ensure data confidentiality, and comply with data protection regulations.
Continuous Monitoring and Maintenance
MDM is an ongoing process that requires continuous monitoring and maintenance to ensure data accuracy and integrity. Organizations should establish processes and procedures to regularly monitor and maintain master data.
Key activities in continuous monitoring and maintenance include:
- Data Auditing: Conducting regular audits of master data to identify data anomalies, inconsistencies, and inaccuracies.
- Data Cleansing and Updates: Performing regular data cleansing and updates to ensure that master data is accurate, complete, and up-to-date.
- Data Governance Compliance: Monitoring and enforcing compliance with data governance policies, data quality standards, and data privacy regulations.
- Data Stewardship: Appointing data stewards responsible for monitoring and maintaining master data, addressing data issues, and ensuring data integrity.
By continuously monitoring and maintaining master data, organizations can ensure that their data remains accurate, consistent, and reliable.
In the next section, we will provide a step-by-step guide to implementing MDM, covering key activities and considerations at each stage.
4. Step-by-Step Guide to Implementing MDM
Implementing Master Data Management requires careful planning, execution, and ongoing maintenance. This step-by-step guide will help organizations navigate the implementation process and ensure MDM success.
Step 1: Assessing Current Data Landscape
The first step in implementing MDM is to assess the current data landscape within the organization. This involves identifying the systems, databases, and applications that store master data and understanding the data quality, data integration, and data governance challenges.
Key activities in this step include:
- Data Inventory: Creating an inventory of systems, databases, and applications that store master data.
- Data Profiling: Analyzing the quality, consistency, and accuracy of master data in the existing systems.
- Data Integration Challenges: Identifying data integration challenges, such as data redundancies, inconsistencies, and data mapping issues.
- Data Governance Assessment: Assessing the existing data governance practices and identifying gaps or areas for improvement.
By conducting a thorough assessment of the current data landscape, organizations can identify key challenges and develop a roadmap for implementing MDM.
Step 2: Defining MDM Objectives and Scope
Once the current data landscape has been assessed, organizations should define their MDM objectives and scope. This involves identifying the key entities to be managed, the desired outcomes, and the scope of the MDM implementation.
Key activities in this step include:
- Identifying Key Entities: Identifying the key entities, such as customers, products, employees, and vendors, that will be managed through MDM.
- Defining Objectives: Defining the objectives and desired outcomes of the MDM implementation, such as improved data quality, operational efficiency, and customer experience.
- Setting Scope: Determining the scope of the MDM implementation, including the systems, databases, and applications that will be included, as well as any specific data integration or data governance requirements.
By clearly defining the MDM objectives and scope, organizations can align their MDM implementation with their business goals and priorities.
Step 3: Selecting the Right MDM Solution
Selecting the right MDM solution is crucial for MDM success. Organizations should evaluate different MDM solutions based on their specific requirements, including data integration capabilities, data governance features, scalability, and ease of use.
Key activities in this step include:
- Vendor Evaluation: Evaluating different MDM solution vendors based on their capabilities, reputation, customer reviews, and industry expertise.
- Requirement Analysis: Analyzing the organization's specific requirements, such as data integration, data governance, and scalability, to determine the best-fit MDM solution.
- Proof of Concept: Conducting proof of concept exercises to validate the capabilities and suitability of the shortlisted MDM solutions.
- Vendor Selection: Selecting the MDM solution vendor that best meets the organization's requirements and budget.
By selecting the right MDM solution, organizations can ensure that their MDM implementation is based on a robust and scalable platform.
Step 4: Data Profiling and Cleansing
Data profiling and cleansing are essential steps in MDM implementation to improve data quality and prepare the data for integration into the MDM system.
Key activities in this step include:
- Data Profiling: Analyzing the quality, consistency, and accuracy of master data in the source systems to identify data issues, anomalies, and inconsistencies.
- Data Cleansing: Removing or correcting data issues, anomalies, and inconsistencies to improve data quality and integrity.
- Data Standardization: Applying standardization rules to ensure consistent data formats, values, and definitions across systems.
- Data Enrichment: Enhancing the data with additional attributes or information to improve its completeness and usefulness.
By profiling and cleansing the data, organizations can ensure that the master data is accurate, consistent, and reliable.
Step 5: Data Integration and Mapping
Data integration and mapping involve bringing together master data from various sources and systems into the MDM system. This step ensures that the master data is consolidated, standardized, and available for use in the MDM system.
Key activities in this step include:
- Data Mapping: Mapping data elements from different source systems to the corresponding master data attributes in the MDM system.
- Data Transformation: Transforming data from different formats and structures to ensure compatibility and consistency within the MDM system.
- Data Loading: Loading transformed data into the MDM system, ensuring that it is accurate, complete, and up-to-date.
- Data Synchronization: Implementing processes to keep the master data in sync with the source systems, ensuring real-time updates and consistency.
By integrating and mapping the data, organizations can eliminate data silos, improve data consistency, and provide a unified view of master data.
Step 6: Establishing Data Governance Policies
Data governance is a crucial aspect of MDM implementation. Establishing data governance policies ensures that master data is managed and maintained according to defined standards and regulations.
Key activities in this step include:
- Data Governance Framework: Establishing a data governance framework that outlines the roles, responsibilities, and processes for managing and maintaining master data.
- Data Quality Standards: Defining data quality standards and metrics to ensure that master data meets predefined criteria.
- Data Privacy and Security Policies: Implementing policies and procedures to protect master data and ensure compliance with data protection regulations.
- Data Governance Council: Creating a data governance council or committee responsible for setting data governance policies, resolving conflicts, and making strategic decisions related to master data.
By establishing robust data governance policies, organizations can ensure that their master data is managed and maintained according to defined standards and regulations.
Step 7: Implementing MDM System and Processes
Implementing the MDM system and processes is a critical step in MDM implementation. This involves configuring the MDM system, setting up data workflows, and implementing data governance processes.
Key activities in this step include:
- MDM System Configuration: Configuring the MDM system based on the organization's requirements, including data models, workflows, and user roles.
- Data Workflows: Defining data workflows that outline the processes and steps for managing and maintaining master data, including data entry, data validation, and data approval.
- Data Governance Processes: Implementing data governance processes, such as data quality monitoring, data issue resolution, and data stewardship.
- Integration with Source Systems: Integrating the MDM system with the source systems to enable real-time data synchronization and updates.
By implementing the MDM system and processes, organizations can establish a robust framework for managing and maintaining master data.
Step 8: Testing and Validation
Testing and validation are essential steps in MDM implementation to ensure that the MDM system and processes are functioning as intended and meeting the organization's requirements.
Key activities in this step include:
- Unit Testing: Testing individual components of the MDM system, such as data models, workflows, and user interfaces, to ensure their functionality and accuracy.
- Integration Testing: Testing the integration between the MDM system and the source systems to ensure data synchronization and consistency.
- User Acceptance Testing: Involving end-users in testing the MDM system and processes to validate their usability, effectiveness, and alignment with business requirements.
- Data Validation: Validating the accuracy, consistency, and integrity of master data in the MDM system against predefined criteria and business rules.
By conducting thorough testing and validation, organizations can ensure that the MDM system and processes meet the organization's requirements and deliver the expected outcomes.
Step 9: Continuous Improvement and Maintenance
MDM is an ongoing process that requires continuous improvement and maintenance to ensure data accuracy, consistency, and integrity.
Key activities in this step include:
- Data Quality Monitoring: Implementing processes to monitor data quality, detect data anomalies, and address data issues in a timely manner.
- Data Cleansing and Updates: Performing regular data cleansing and updates to ensure that master data remains accurate, complete, and up-to-date.
- Data Governance Compliance: Continuously monitoring and enforcing compliance with data governance policies, data quality standards, and data privacy regulations.
- Data Stewardship: Appointing data stewards responsible for monitoring and maintaining master data, addressing data issues, and ensuring data integrity.
By continuously monitoring and maintaining master data, organizations can ensure that their data remains accurate, consistent, and reliable.
In the next section, we will discuss best practices for MDM implementation that can help organizations achieve MDM success.
5. Best Practices for MDM Implementation
Implementing Master Data Management requires a strategic and well-planned approach. Organizations should consider the following best practices to ensure MDM success:
Executive Sponsorship and Stakeholder Engagement
MDM initiatives require strong executive sponsorship and stakeholder engagement. The support and involvement of senior leaders are crucial for securing resources, driving organizational change, and ensuring the success of the MDM implementation.
Key activities in this best practice include:
- Executive Sponsorship: Appointing an executive sponsor who will champion the MDM initiative, communicate its importance, and provide guidance and support throughout the implementation process.
- Stakeholder Engagement: Engaging key stakeholders, including business leaders, IT teams, data owners, and end-users, to gather requirements, address concerns, and ensure alignment with business goals.
- Communication and Training: Providing regular communication and training sessions to keep stakeholders informed about the MDM initiative, its progress, and its impact on the organization.
By securing executive sponsorship and engaging stakeholders effectively, organizations can ensure that the MDM initiative receives the necessary support and resources for success.
Cross-Functional Collaboration
Successful MDM implementation requires cross-functional collaboration and alignment. MDM initiatives often involve multiple departments and business functions, and collaboration is essential for ensuring data consistency, defining data governance policies, and implementing data workflows.
Key activities in this best practice include:
- Cross-Functional Team: Establishing a cross-functional team comprising representatives from different departments and business functions to provide input, share expertise, and drive the MDM implementation.
- Regular Collaboration Meetings: Conducting regular collaboration meetings to discuss data governance policies, data quality issues, and data integration challenges, and to align on the implementation roadmap.
- Data Stewardship Collaboration: Promoting collaboration among data stewards from different departments to ensure consistent data governance practices, resolve data issues, and share best practices.
By fostering cross-functional collaboration, organizations can create a unified approach to MDM, ensuring that data is managed consistently and effectively across the organization.
Change Management and Training
MDM implementation often involves significant organizational change, and effective change management is crucial for successful adoption and implementation.
Key activities in this best practice include:
- Change Impact Assessment: Assessing the impact of MDM implementation on different departments, processes, and roles, and identifying potential challenges and resistance to change.
- Communication and Education: Communicating the benefits of MDM implementation, the reasons for change, and the expected outcomes to all stakeholders. Providing training and education to ensure that stakeholders understand their roles and responsibilities in the MDM initiative.
- Change Champions: Appointing change champions within different departments to foster adoption, address concerns, and promote the benefits of MDM.
By effectively managing change and providing comprehensive training and education, organizations can ensure smooth adoption of MDM and maximize its benefits.
Data Stewardship and Ownership
Data stewardship and ownership are critical for MDM success. Data stewards play a key role in managing and maintaining master data, resolving data issues, and ensuring data integrity.
Key activities in this best practice include:
- Data Steward Roles and Responsibilities: Defining roles and responsibilities of data stewards, including data quality monitoring, data issue resolution, and data governance compliance.
- Data Steward Training: Providing training and education to data stewards to ensure that they have the necessary skills and knowledge to perform their roles effectively.
- Data Ownership: Establishing data ownership within different departments and business functions to ensure accountability and responsibility for managing data.
By establishing clear roles and responsibilities for data stewards and promoting data ownership, organizations can ensure that master data is managed effectively and consistently.
Scalability and Flexibility
MDM implementation should be scalable and flexible to accommodate future growth, changing business requirements, and evolving data landscapes.
Key activities in this best practice include:
- Scalable Architecture: Designing an MDM architecture that can scale with the organization's growth, handle increasing data volumes, and support future integration with new systems and applications.
- Flexibility in Data Models: Designing data models that are flexible and adaptable to changing business requirements, allowing for the addition of new attributes, entities, and relationships as needed.
- Modular Approach: Adopting a modular approach to MDM implementation, allowing for incremental updates, enhancements, and integration with new systems or data sources.
By ensuring scalability and flexibility in MDM implementation, organizations can future-proof their MDM initiatives and adapt to changing business needs and data landscapes.
In the next section, we will discuss the challenges and pitfalls that organizations may encounter during MDM implementation and provide strategies for overcoming them.
6. Challenges and Pitfalls in MDM Implementation
Implementing Master Data Management can present various challenges and pitfalls that organizations need to be aware of and address proactively to ensure MDM success.
Lack of Data Governance
One of the common challenges in MDM implementation is the lack of data governance. Without robust data governance policies and processes, organizations may struggle to establish data standards, resolve conflicts, and ensure data consistency and integrity.
To overcome this challenge, organizations should establish a data governance framework, define data governance policies, and appoint data stewards who are responsible for managing and maintaining master data.
Data Quality Issues
Data quality issues can undermine the success of MDM implementation. Poor data quality can lead to incorrect insights, inefficient operations, and poor decision-making.
To address data quality issues, organizations should implement data profiling and cleansing activities, establish data quality standards, and regularly monitor data quality to identify and resolve data issues.
Resistance to Change
Resistance to change is another challenge that organizations may face during MDM implementation. Employees may be resistant to adopting new processes, technologies, or roles related to MDM.
To overcome resistance to change, organizations should communicate the benefits of MDM implementation, involve stakeholders in the decision-making process, provide training and education, and establish change champions to promote adoption and address concerns.
Legacy System Integration
Integrating MDM with legacy systems can be challenging due to compatibility issues, data mapping complexities, and technical limitations.
To address legacy system integration challenges, organizations should conduct a thorough assessment of the existing systems, define data integration requirements, and work closely with IT teams to ensure seamless integration and data synchronization.
Scalability and Complexity
MDM implementation can become complex and challenging to scale, especially in organizations with multiple business units, regions, or systems.
To address scalability and complexity challenges, organizations should design a scalable MDM architecture, adopt a modular approach to implementation, and ensure flexibility in data models to accommodate future growth and changing business requirements.
By addressing these challenges proactively and implementing appropriate strategies, organizations can overcome the pitfalls and ensure MDM success.
In the next section, we will explore the relationship between MDM and Customer 360, highlighting the benefits of MDM in achieving a comprehensive view of customers.
7. MDM and Customer 360
Customer 360 is a holistic view of customer data that encompasses all interactions, transactions, and preferences of a customer across multiple touchpoints and channels. MDM plays a crucial role in achieving a comprehensive and accurate Customer 360 view.
Customer Data Integration
Customer Data Integration (CDI) is a key component of MDM that focuses on consolidating and integrating customer data from various sources to create a single, unified view of each customer.
CDI involves:
- Data Matching and Deduplication: Identifying and resolving duplicate customer records from different systems to ensure a single customer view.
- Data Enrichment: Enhancing customer data with additional attributes, such as demographics, purchase history, and preferences, to gain a deeper understanding of each customer.
- Data Synchronization: Establishing processes to keep the customer data in sync across different systems, ensuring real-time updates and consistency.
By integrating customer data through CDI, organizations can achieve a comprehensive and accurate Customer 360 view.
Single Customer View
MDM enables the creation of a single, unified view of each customer, regardless of how the data is captured or stored. This single customer view consolidates customer data from multiple systems, databases, and channels, providing a holistic understanding of each customer's interactions, preferences, and history.
The single customer view allows organizations to:
- Personalize Customer Experiences: With a comprehensive understanding of each customer, organizations can personalize interactions, tailor marketing campaigns, and deliver targeted offers and recommendations.
- Improve Customer Service: By having access to a unified view of customer data, organizations can provide better customer service, resolve issues more efficiently, and offer tailored solutions.
- Enhance Customer Analytics: The single customer view provides a rich source of data for customer analytics and insights, enabling organizations to identify trends, preferences, and opportunities for cross-selling or upselling.
By achieving a single customer view through MDM, organizations can deliver personalized experiences, improve customer service, and gain valuable insights into customer behavior.
Personalization and Customer Experience
MDM enables organizations to personalize customer experiences by accurately identifying customers across different touchpoints and channels. With a unified view of customer data, organizations can deliver targeted marketing campaigns, tailored product recommendations, and personalized customer service.
Personalization and customer experience efforts supported by MDM include:
- Segmentation: Dividing customers into distinct segments based on their demographics, preferences, or behaviors to deliver targeted marketing messages and offers.
- Omnichannel Experience: Providing a seamless and consistent experience across multiple touchpoints and channels, enabling customers to interact with the organization through their preferred channels.
- 360-Degree Customer Profiles: Creating comprehensive customer profiles that include contact information, purchase history, preferences, and interactions, ensuring a holistic understanding of each customer.
By leveraging MDM for personalization and customer experience, organizations can build stronger customer relationships, enhance customer loyalty, and drive business growth.
Customer Analytics and Insights
MDM provides a solid foundation for customer analytics and insights. By consolidating and integrating customer data, organizations can gain valuable insights into customer behavior, preferences, and trends.
Key benefits of MDM in customer analytics include:
- 360-Degree View: MDM enables organizations to create a comprehensive view of each customer, allowing for deeper insights and understanding.
- Entity resolution: A critical aspect of MDM that involves identifying and linking related data records to create a unified and accurate view of entities, such as customers, products, or locations.
- Data Consistency: With consistent and accurate customer data, organizations can perform more reliable and accurate customer analytics.
- Data Integration: MDM integrates customer data from various sources, enabling organizations to perform cross-channel analysis and identify patterns and trends.
- Segmentation and Targeting: MDM enables organizations to segment customers based on their attributes and behaviors, allowing for targeted marketing campaigns and personalized offerings.
By leveraging MDM for customer analytics and insights, organizations can uncover valuable trends, patterns, and opportunities for driving growth and improving customer satisfaction.
In the next section, we will explore future trends in Master Data Management, including the impact of artificial intelligence, cloud-based solutions, and emerging technologies.
8. Future Trends in Master Data Management
Master Data Management (MDM) is continually evolving in response to technological advancements and shifting business requirements. Several future trends are influencing the MDM landscape and offering new opportunities for organizations.
Artificial Intelligence and Machine Learning
Artificial Intelligence (AI) and Machine Learning (ML) are playing a pivotal role in transforming MDM by automating various data management processes, thereby enhancing data quality and accuracy.
- Data Matching and Deduplication: AI and ML algorithms are capable of accurately identifying and resolving duplicate records, thereby bolstering data quality and integrity.
- Data Enrichment: AI and ML technologies facilitate the automation of enriching customer data with additional attributes, such as sentiment analysis, social media data, and behavioral data.
These applications of AI and ML in MDM are revolutionizing the way organizations manage their master data, leading to more efficient and effective data management processes.
9. Case Studies: MDM Success Stories
Master Data Management (MDM) played a pivotal role in streamlining operations and enhancing efficiency for a global telecommunications company. By building golden records of customers within the data warehouse, the company achieved a unified and accurate view of customer data across various systems and channels. This enabled the organization to eliminate data silos, reduce redundancy, and ensure consistent and reliable customer information for all business operations.
The implementation of MDM led to improved customer service, targeted marketing strategies, and optimized sales processes. The golden records facilitated better decision-making by providing a single source of truth for customer data, leading to enhanced operational agility and a significant reduction in errors. Moreover, the streamlined data management process allowed for quicker response times to customer inquiries and improved overall customer satisfaction.
By leveraging MDM to build golden records of customer data, the company experienced a marked improvement in operational efficiency, enabling them to make data-driven decisions and deliver personalized experiences to their customers across all touchpoints.
10. Conclusion: Unlocking the Power of Master Data
In addition to the rapidly evolving fields of artificial intelligence (AI) and machine learning (ML), cloud-based solutions are also playing a pivotal role in shaping the future of Master Data Management (MDM). The advent of cloud-based MDM platforms has revolutionized the way organizations handle their master data, offering unparalleled scalability, flexibility, and cost-effectiveness. With the ability to easily manage and access their master data from anywhere, organizations are empowered to make informed decisions and drive strategic initiatives with ease.
Moreover, the landscape of MDM is constantly evolving, with emerging technologies like blockchain garnering significant attention for their potential to enhance data security and integrity within MDM systems. Blockchain, a decentralized and immutable ledger technology, holds promise in ensuring the trustworthiness and reliability of master data, thereby bolstering the overall effectiveness of MDM processes. By leveraging blockchain, organizations can establish a transparent and tamper-proof system for managing their critical data assets.
These advancements in MDM, fueled by the convergence of AI, ML, and cloud-based solutions, are poised to drive innovation and deliver valuable insights for strategic decision-making. The scalability and flexibility offered by cloud-based MDM platforms enable organizations to seamlessly adapt to changing business requirements and scale their operations as needed. Furthermore, the cost-effectiveness of cloud-based solutions allows organizations to optimize their resource allocation and invest in other critical areas of their business.
As organizations continue to embrace the power of AI, ML, and cloud-based solutions in their MDM strategies, they are well-positioned to unlock the full potential of their master data. By harnessing the capabilities of these cutting-edge technologies, organizations can gain a competitive edge in the market, drive operational efficiency, and deliver enhanced customer experiences. The future of MDM is undoubtedly bright, with ongoing advancements paving the way for a data-driven revolution that will shape industries and empower organizations to thrive in the digital age.