Somewhere along the line, folks began defining data engineers as people who worked in specific technologies like Hadoop or Spark. From there, they gained a reputation for being super complex and technical.
While they can be both of those 👆 things, that doesn’t mean analysts and business users should just ignore everything data engineering-related. Sure, technology changes, but the goal of data engineers remains the same: To turn raw, messy, complex data into high-quality information other teams can use. And because engineering makes the whole data machine run, understanding the lifecycle data moves through makes you a better data collaborator – regardless of your role.
What is a data engineering lifecycle?
The data engineering lifecycle is a method for overseeing data engineering processes including data acquisition, integration, storage, processing, and analysis. This lifecycle incorporates structured and interconnected stages aimed at consistently delivering high-quality data engineering projects. The main objective is to assist data engineers in creating reliable, high-quality data sets that are suitable for their intended use and can aid in business decision-making.
To help folks better understand the data engineering lifecycle, Matt Housley – co-founder of Ternary Data and co-author of the book Fundamentals of Data Engineering – broke it down during his Summer Community Days session titled, How Understanding the Data Engineering Lifecycle Helps Us All Work Better with Data Engineers.
In his talk, Matt split the data engineering lifecycle problems into 🔑 stages (generation, storage, ingestion, transformation, and serving). Plus, as a teaser (if you haven’t read this 💎 already), he discussed concepts from the Fundamentals of Data Engineering to introduce these stages to data practitioners of all flavors, to help them better collaborate with data engineers to deliver outstanding data products.
Data engineering challenges
Matt started out by tackling the two biggest challenges currently facing data engineers: Communication and holistic thinking.
For starters, communication needs to flow both ways. ↔️ Engineers need to clearly express what’s happening to data, and stakeholders need to clearly express what raw data is or what finished data should look like. 🗣
“We need communication to make sure data engineers understand what the data is before they turn it into a useful product, and to make sure those products are the right things,” Matt explained. “If you can become a better communicator as a stakeholder or as an engineer, you’ll be way more successful in delivering results.”
Holistic thinking, on the other hand, means getting past the technology and considering the big picture. That’s where understanding the data engineering lifecycle comes in clutch. 🔁
“We need to think about where data starts, where it ends up, how it flows through the pipeline, and how we maintain quality as it moves,” Matt said. “That’s what a ‘holistic view of data’ means.”
The data engineering lifecycle
In “Fundamentals of Data Engineering,” Matt and his co-author, Joe Reis, introduced the lifecycle concept. Essentially, the lifecycle breaks the data pipeline we all know and love into its critical pieces to see where data starts and how we maintain quality as it moves to the end.
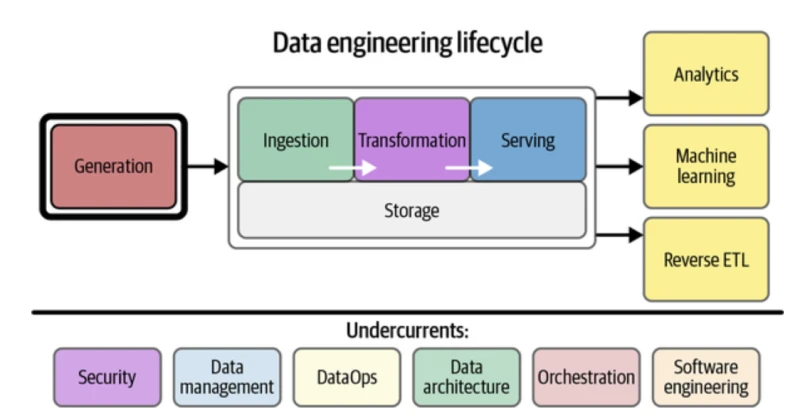
Generation
In the first stage of the lifecycle, data is born. 🐣 It’s created in a variety of platforms, and data engineers rarely have control over any of them. The engineers need to communicate with app developers and platform experts to understand what’s being created.
At this stage, the data “works” only within its source platform. It’s not yet ready for consumption by operational analytics, BI, machine learning, reverse ETL, or any other application.
Ingestion
In the next stage, the raw data moves into the engineer’s pipeline. It’s still messy, but now it’s in a place where we can clean it up. 🧼
Transformation
In the transformation stage, data engineers start working with the data. It gets modeled, filtered, and joined. Depending on the desired result, engineers might start working with statistics and aggregations. Our homely little data caterpillar is quickly becoming a butterfly. 🦋
Serving
In the final stage of the data engineering lifecycle, the data – transformed into useful information – gets served up to the end user. 🍽️ It might be delivered to a dashboard, a data science team, or a BI platform.
Undercurrents flow across lifecycle stages
Six foundational data engineering concepts flow across the stages of the lifecycle. Engineers need to keep these undercurrents in mind no matter what stage of the pipeline they’re working with.
- Security. As data pros, we can never forget the importance of security. At every stage of the data engineering lifecycle, we need to keep private data private and protected from misuse.
- Data management. Matt defines this as best practices like governance, maintaining data quality, and keeping track of what data is collected and where it’s stored.
- DataOps. DataOps is the integrated approach that takes concepts from DevOps and applies them to data.
- Data architecture. We’re not just talking about individual technologies that come and go. This is the big picture of how data gets processed and flows through systems.
- Orchestration. Orchestrating data means coordinating all the moving parts of the lifecycle.
- Software engineering. OK, yes, data engineers aren’t defined by their tools, but they do need to be proficient in the pipeline’s technology.
You don’t get data science without data engineering
A few years back, Monica Rogati proposed the data science hierarchy of needs, which has since been cited all over the Internet. Up at the top are functions like AI and deep learning – the glamorous things that keep data scientists on those “sexiest jobs” lists.
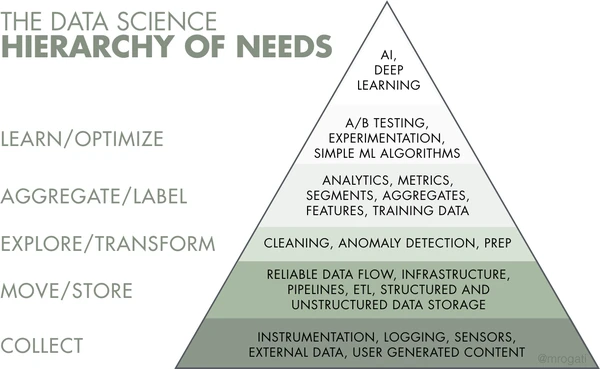
But you can’t get there without a solid foundation of less-sexy layers like instrumentation, infrastructure, and anomaly detection.
“Everyone wants to deliver a really awesome dashboard or a model to transcribe speech or a live operational analytics dashboard for situational awareness,” Matt said. “But to do that, you have to have these layers underneath and you have to have communication between the different layers.”
Even if you have every layer of the pyramid in place, Matt said, if the data engineers in the basement don’t communicate the awesome things they’re doing with the analysts halfway up, and the analysts don’t tell the engineers what kind of insights they want to create, you won’t successfully execute the functions at the top.
So, despite its reputation, data engineering is not a mystical art. 🪄 Nor is it simply being really, really good at Airflow. It’s the mechanism that allows data science to produce the amazing insights we’re all aiming for.
And the better analysts and other stakeholders understand how data engineering works, the better they’ll work with engineers and get the results they’re after.
Want to learn more? Check out Matt’s full presentation on the data engineering lifecycle from our Summer Community Days here. 👈
✨ Then head over to the Operational Analytics Club to share your view and join the conversation.